diff --git a/Software_Carbon_Efficiency_Rating(SCER)/CLA.md b/CLA.md
similarity index 100%
rename from Software_Carbon_Efficiency_Rating(SCER)/CLA.md
rename to CLA.md
diff --git a/README.md b/README.md
index 585aec4..f910dee 100644
--- a/README.md
+++ b/README.md
@@ -39,7 +39,7 @@ Carbon Efficiency: The amount of carbon dioxide equivalent (CO2e) emitted per US
### Meeting Details
- Bi-weekly on a Wednesday @ 09:00 PT / 1700 BST
-- [Become a member](https://wiki.greensoftware.foundation/orientation/signup)
+- [Become a member](https://wiki.greensoftware.foundation/register)
### Software Carbon Efficiency Rating
- [Current baseline](https://github.com/Green-Software-Foundation/scer)
diff --git a/Resources/events.md b/Resources/events.md
deleted file mode 100644
index fe97af1..0000000
--- a/Resources/events.md
+++ /dev/null
@@ -1,4 +0,0 @@
-Upcoming events for participation:
-- [Open Source Summit Japan](https://events.linuxfoundation.org/open-source-summit-japan/)
-- [Open Source Summit Asia](https://events.linuxfoundation.org/kubecon-cloudnativecon-open-source-summit-ai-dev-china/)
-- [Gosim China 2024](https://china2024.gosim.org/)
diff --git a/Resources/slide_decks.md b/Resources/slide_decks.md
deleted file mode 100644
index 8076c3e..0000000
--- a/Resources/slide_decks.md
+++ /dev/null
@@ -1,13 +0,0 @@
-List SCER presentation and resources here:
-- [Advancing Responsible AI: Unveiling the Software Carbon Efficiency Rating (SCER) for AI Models (PDF and Youtube Video)](https://aideveu24.sched.com/event/1c1lg?iframe=no) at LF AI_dev: Open Source GenAI & ML Summit Europe 2024
-
-
-
-
-
-
-References:
-- NutriScore: https://en.wikipedia.org/wiki/Nutri-Score
-- EnergyGuide: https://en.wikipedia.org/wiki/EnergyGuide
-- EnergyStar: https://www.energystar.gov/
-- ISO 9000, Quality Management Standard Framework: https://www.iso.org/standards/popular/iso-9000-family
diff --git a/SCER_Certification_Program/Reporting.md b/SCER_Certification_Program/Reporting.md
deleted file mode 100644
index c9acbe8..0000000
--- a/SCER_Certification_Program/Reporting.md
+++ /dev/null
@@ -1,3 +0,0 @@
-### Report SCER Usage Here (Organization Name, Conformance Levels, References):
-Example:
-- GSF, Level 2, https://greensoftware.foundation/
diff --git a/SCER_Certification_Program/SCER_Certification_Prorgram_for_LLMs.md b/SCER_Certification_Program/SCER_Certification_Prorgram_for_LLMs.md
deleted file mode 100644
index 3e82ed2..0000000
--- a/SCER_Certification_Program/SCER_Certification_Prorgram_for_LLMs.md
+++ /dev/null
@@ -1,50 +0,0 @@
-### SCER Certification Program for LLMs
-
-**Introduction:**
-The SCER (Software Carbon Efficiency Rating) certification program for Large Language Models (LLMs) aims to promote transparency, accountability, and environmental responsibility in the development and deployment of AI technologies. The program is inspired by successful initiatives like [NutriScore](https://www.santepubliquefrance.fr/en/nutri-score), [Energy Star](https://www.energystar.gov/), and [EnergyGuide](https://consumer.ftc.gov/articles/how-use-energyguide-label-shop-home-appliances), providing a clear and standardized framework for assessing and communicating the carbon efficiency of LLMs.
-
-**Certification Levels:**
-The SCER certification program has two levels:
-
-**Level 1. SCER Process Conformance Certification**
- - **Description:** This certification level ensures that organizations adhere to the SCER standardized framework and complete the four-step process.
- - **Instructions:**
- 1. Complete the four-step process outlined by [SCER for LLMs](https://github.com/chrisxie-fw/scer/blob/Dev/use_cases/SCER_FOR_LLM/SCER_For_LLM_Specification.md):
- - **Step 1:** LLMs Categorization
- - **Step 2:** Carbon Benchmarking
- - **Step 3:** Rating
- - **Step 4:** Visuals and Labelling
- 2. Report usage and conformance to the [SCER Working Group (WG)](Reporting.md) within the Green Software Foundation (GSF).
- 3. Use the SCER Process Conformance Label
- - **Outcome:** Organizations meeting these criteria are granted the right to display the SCER Process Conformance label on their products or services.
- - **Label:**
-
-
-
- (Experimental label image)
-
-**Level 2. SCER Rating Certification**
- - **Description:** This certification level assesses the actual carbon efficiency rating of the LLMs and provides a rating based on the SCER framework. Achieving this certification also implies compliance with the Level 1 SCER Process Conformance Certification.
- - **Instructions:**
- 1. Use the SCER for LLMs process to obtain a carbon efficiency rating.
- 2. Display the rating on the relevant products and services.
- 3. Report usage and conformance to the [SCER WG in GSF](Reporting.md).
- - **Outcome:** Organizations meeting these criteria are granted the right to display the SCER Rating label on their products or services.
- - **Label:**
-
-
-
- (Experimental label image)
-
-**Reporting Requirements:**
-- Both certification levels require organizations to report their usage and conformance to the SCER WG in the GSF.
-- Organizations are encouraged to voluntarily report any changes when their conformance status is altered.
-
-**Standards and Compliance:**
-- SCER standards are periodically reviewed and updated to reflect technological advancements and market changes, ensuring the label remains a mark of best practices for high carbon efficiency.
-- The SCER WG monitors compliance through ongoing testing and market surveillance.
-- Products or services found to be non-compliant can have their certification revoked.
-
-**Summary:**
-
-The SCER certification program for LLMs is a comprehensive initiative designed to encourage sustainable practices in AI development. By providing clear guidelines and rigorous standards, SCER aims to reduce the carbon footprint of LLMs and promote environmental responsibility within the AI industry.
diff --git a/SCER_Certification_Program/images/SCER_Label.webp b/SCER_Certification_Program/images/SCER_Label.webp
deleted file mode 100644
index fe10132..0000000
Binary files a/SCER_Certification_Program/images/SCER_Label.webp and /dev/null differ
diff --git a/SCER_Certification_Program/images/SCER_Rating_Label.webp b/SCER_Certification_Program/images/SCER_Rating_Label.webp
deleted file mode 100644
index 5947732..0000000
Binary files a/SCER_Certification_Program/images/SCER_Rating_Label.webp and /dev/null differ
diff --git a/SCER_Certification_Program/images/scer_process_conformance.png b/SCER_Certification_Program/images/scer_process_conformance.png
deleted file mode 100644
index 30bad43..0000000
Binary files a/SCER_Certification_Program/images/scer_process_conformance.png and /dev/null differ
diff --git a/SCER_Certification_Program/images/scer_rating_conformance.png b/SCER_Certification_Program/images/scer_rating_conformance.png
deleted file mode 100644
index a16349a..0000000
Binary files a/SCER_Certification_Program/images/scer_rating_conformance.png and /dev/null differ
diff --git a/SPEC.md b/SPEC.md
new file mode 100644
index 0000000..1011226
--- /dev/null
+++ b/SPEC.md
@@ -0,0 +1,195 @@
+---
+version: 0.0.1
+---
+# Software Carbon Efficiency Rating (SCER) Specification
+
+## Introduction
+
+
+In the context of global digital transformation, the role of software in contributing to carbon emissions has become increasingly significant. This necessitates the development of standardized methodologies for assessing the environmental impact of software systems.
+
+Rationale:
+
+- The Rising Carbon Footprint of Software: The digitization of nearly every aspect of modern life has led to a surge in demand for software solutions, subsequently increasing the energy consumption and carbon emissions of the IT sector.
+- The Need for a Unified Approach: Currently, the lack of a standardized system for labeling the carbon efficiency of software products hinders effective management and reduction of carbon footprints across the industry.
+
+This document aims to establish the Software Carbon Efficiency Rating (SCER) Specification, a standardized framework for labeling the carbon efficiency of software systems. The SCER Specification aims to serve as a model for labeling software products according to their Software Carbon Intensity (SCI), and it is adaptable for different software categories.
+
+
+## Scope
+
+This specification provides a framework for displaying, calculating, and verifying software carbon efficiency labels. By adhering to these requirements, software developers and vendors can offer consumers a transparent and trustworthy method for assessing the environmental impact of software products.
+
+It outlines the label format, presentation guidelines, display requirements, computation methodology used to determine the software's carbon efficiency, and the requirements for providing supporting evidence to demonstrate the accuracy of the carbon efficiency claims.
+
+This specification is intended for a broad audience involved in the creation, deployment, or use of software systems, including but not limited to:
+- Software developers
+- IT professionals
+- Policy-makers
+- Business leaders
+
+
+## Normative references
+
+ISO/IEC 21031:2024
+Information technology — Software Carbon Intensity (SCI) specification.
+
+ISO/IEC 40500
+Information technology — W3C Web Content Accessibility Guidelines (WCAG) 2.0.
+
+ISO/IEC 18004:2024
+Information technology — Automatic identification and data capture techniques — QR code bar code symbology specification.
+
+## Terms and definitions
+
+For the purposes of this document, the following terms and definitions apply.
+
+ISO and IEC maintain terminological databases for use in standardization at the following addresses:
+- ISO Online browsing platform: available at https://www.iso.org/obp
+- IEC Electropedia: available at http://www.electropedia.org/
+
+> [!NOTE]
+> TODO: Update these definitions
+
+- Software Application: TBD
+- Software Carbon Efficiency: TBD
+- Software Carbon Intensity: TBD
+- Carbon: TBD
+- Functional Unit: TBD (From SCI)
+- Manifest File: TBD
+- QR Code: TBD
+
+
+The following abbreviations are used throughout this specification:
+
+> [!NOTE]
+> TODO: Update these abbreviations
+- SCI
+- SCER
+
+> [!NOTE]
+> For ease of ref, removed in final spec.
+> - Requirements – shall, shall not
+> - Recommendations – should, should not
+> - Permission – may, need not
+> - Possibility and capability – can, cannot
+
+## Core Requirements
+
+### Ease of understanding
+A label that is hard to understand or requires expertise unavailable to most software consumers would defeat the purpose of adding transparency and clarity.
+
+SCER labels shall be uncluttered and have a clear and simple design that Shall be easily understood.
+
+### Ease of Verification
+The SCER label Shall make it easy for a consumer of a software application to verify any claims made.
+
+Consumers should have all the information they need to verify any claims made on the label and to ensure the underlying calculation methodology or any related specification has been followed accurately.
+
+### Accessible
+The SCER label and format shall be accessible to and meet accessibility specifications.
+
+### Language
+The SCER label Should be written using the English language and alphabet.
+
+## Calculation Methodology
+
+The SCI shall be used as the calculation methodology for the SCER label.
+
+Any computation of a SCI score for the SCER label SHALL adhere to all requirements of the SCI specification.
+
+> Note: SCI is a Software Carbon Efficiency Specification computed as an "Carbon per Functional Unit" of a software product. For example, Carbon per Prompt for a Large Language Model.
+
+## Presentation Guidelines
+
+Components of a SCER Label
+
+### SCI Score:
+The presentation of the SCI score Shall follow this template
+
+`[Decimal Value] gCO2eq per [Functional Unit]`
+
+- Where `[Decimal Value]` is the SCI score itself
+- Where the common term `Carbon` Shall be used to represent the more technical term `CO2e` (Carbon Dioxide Equivalent)
+- The symbol `/` Shall Not be used in place of `Per`
+- Where `[Functional Unit]` is text describing the Functional Unit as defined in the SCI calculation for this software application
+
+### SCI Version
+The SCI version Should be visible, even in small sizes.
+
+The SCI version Shall describe which version of the SCI specification this SCER label complies with and have the following format:
+
+`[Short Name] [Version]`
+
+- Where `[Short Name]` is the abbreviated version of the SCI specification this SCER label is representing
+- Where `[Version]` is the official SCI specification version this label refers to.
+
+For example:
+- `SCI 1.1`
+- `SCI AI 1.0`
+
+### QR Code
+The QR Code Shall be a URL represented as a QR code as per ISO/IEC 18004:2024
+
+The URL Shall point to a publicly accessible website where you can download a manifest file that meets the requirements in the *Supporting Evidence Section*
+
+The URL Shall Not require a login, and it Shall be publicly accessible by anonymous users or non-human automated bots/scripts.
+
+## Display Requirements
+
+The SCER Label Shall conform to this layout:
+
+> [!NOTE]
+> This is a WIP, the current design doesn't meet size flexibilty requirements.
+
+
+
+- Color: ??
+- Size: ??
+- Placement: ??
+- Font: ??
+- Example: ??
+
+## Supporting Evidence
+Per the presentation guidelines, the SCER label will link to a manifest file that provides evidence to support any claims made on the label.
+
+The manifest file Shall meet three criteria to pass as supporting evidence.
+
+### Conformance
+Evidence that the underlying SCI requirements have been met in the computation of the SCI score.
+
+The Manifest File Shall clearly describe the Software Boundary per the SCI specification.
+
+The Manifest File Shall follow the Impact Manifest Protocol Standard for communicating environmental impacts.
+
+The Manifest File should use granular data that aligns with SCI recommendations.
+
+### Correctness
+Correctness is confirming the numbers in the manifest file match the information on the SCER label.
+
+The Manifest File shall have an aggregate value for the SCI score that matches the reported score on the SCER label.
+
+The Manifest File shall have a Functional Unit that matches the reported Functional Unit on the SCER label.
+
+### Verification
+Verification is the act of confirming the evidence supports the claim.
+
+Verification of a SCER label Shall be possible using open source software and open data.
+
+Verification Shall be free for the end user and Shall Not require purchasing licenses for software or data or logging into external systems.
+
+If Verification requires access to data, that data Shall also be publicly available and free to use.
+
+
+### 4. Appendices
+
+Supporting documents, example calculations, and reporting templates.
+
+---
+
+### 5. References
+
+List of references used in the creation of the SCER Specification.
+
+---
+
diff --git a/Software_Carbon_Efficiency_Rating(SCER)/Discussions.md b/Software_Carbon_Efficiency_Rating(SCER)/Discussions.md
deleted file mode 100644
index 88f4b75..0000000
--- a/Software_Carbon_Efficiency_Rating(SCER)/Discussions.md
+++ /dev/null
@@ -1,49 +0,0 @@
-## Discussions
-1. Different standard rating programs call for different rating scope, calculation, and algorithm, etc. Some represent the ratings using number scores (e.g. EnergyStar), some using alphabetic letters (NutriScore). Therefore, in order for SCER to be widely adopted, and be flexible enough to fit a wide range of use case scenarios, the design of the SCER spec shall adopt the generic and modular approach for standardization, and enable domain experts from different industry verticals to define category-specific SCER standard, based on the generic SCER spec.
-2. SCI addressed the score part, but lacking **relative rating specification**. Therefore, SCER is supplementing SCI spec to provide an easy-to-understand rating mechanism. SCER also defines the standard specification for software categorization, benchmark definition, and rating definition. These are used as guidelines for users of SCER spec to create their own category-specific SCER specifications.
-2. With respect to the use of SCER spec, it involves data collection and publication of the ratings for various types or categories of software. For instance, refer to [MLPerf](https://mlcommons.org/) and [Geekbench](https://www.geekbench.com/), both of which defined a standard set of workload, in the case of MLPerf, the workload is open sourced, in the case of Geekbench, the workload is close sourced. Both of them provide a standard set of workload and benchmarks. They also provide a means of data collection and intuitive result publication. What are the directions of SCER in this respect? Do we want to define the spec so that other people can use it to define their own category specific SCER spec, or do we create a SCER plaform that's more like MLPerf or Geekbench where benchmarks and data collection of workload are crowd-sourced, and the results are published on a central SCER platform?
-1. House-keeping item: Confirming CLA (Contributor License Agreements) handling:
- - GitHub Integration: There are tools like CLA assistant that integrate with GitHub to manage CLA confirmations. When a contributor submits a pull request, they are prompted to agree to the CLA with a single click if they haven't done so already for that project.
- - Git Signed-off-by Statement: In certain projects, especially those using Git, a contributor might use the signed-off-by statement in their commits as an indication that they agree to the terms of the CLA, though this method is less formal and typically used in conjunction with another method.
-
-
-## References
-This References section demonstrates cases where different rating, labelling systems are used for different use cases and industry verticals.
-
-### EnergyStar:
-
-To earn the ENERGY STAR, eligible commercial buildings must earn an 1–100 ENERGY STAR score of 75 or higher—indicating that they operate more efficiently than at least 75% of similar buildings nationwide. Before applying, a building's application must be verified by a Professional Engineer or Registered Architect.
-
-
-
-
-
-### Nutri-Score:
-Nutri-Score is a front-of-package nutritional label that converts the nutritional value of food and beverages into a simple overall score. It is based on a scale of five colors and letters:
-- A: Green to represent the best nutritional quality
-- B: Light green, meaning it's still a favorable choice
-- C: Yellow, a balanced choice
-- D: Orange, less favorable
-- E: Dark orange to show it is the lowest
-
-The Nutri-Score calculation pinpoints the nutritional value of a product based on the ingredients. It takes into account both positive points (fiber content, protein, vegetables, fruit, and nuts) and negative points (kilojoules, fat, saturated fatty acids, sugar, and salt).
-
-The Nutri-Score is calculated per 100g or 100ml. The goal of the Nutri-Score is to influence consumers at the point of purchase to choose food products with a better nutritional profile, and to incentivize food manufacturers to improve the nutritional quality of their products.
-
-
-
-### CDP:
-[CDP](https://www.cdp.net/en/info/about-us) (formerly Carbon Disclosure Project): A global disclosure system for companies, cities, states, and regions to manage their environmental impacts.
-- Data collection as a form of company disclosure: CDP provides guide that covers the key steps to disclose as a company including setting up a CDP account, responding to the CDP questionnaire(s), and receiving a CDP score.
-- A CDP score is a snapshot of a company’s environmental disclosure and performance. CDP's scoring methodology is fully aligned with regulatory boards and standards, and provides comparability in the market.
-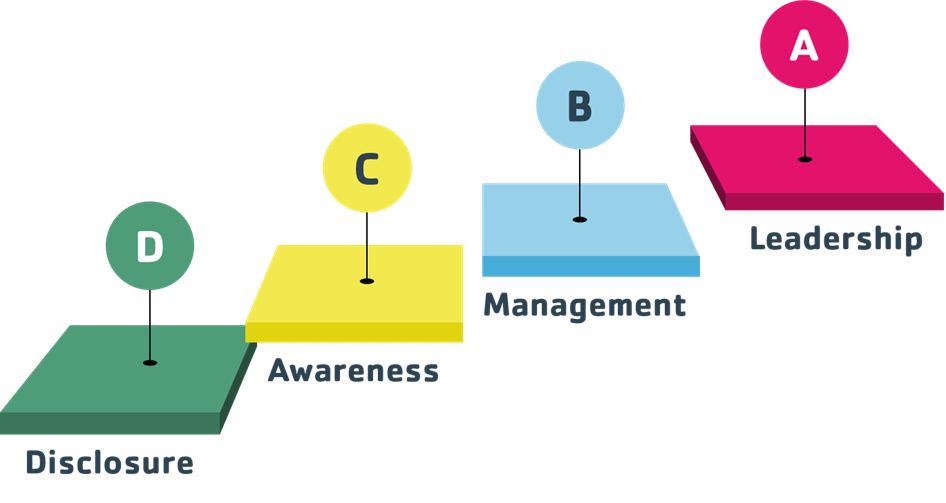
-
-
-### LEED
-LEED stands for Leadership in Energy and Environmental Design. It is the most widely used green building rating system in the world. LEED is an environmentally oriented building certification program run by the U.S. Green Building Council (USGBC).
-LEED provides a framework for healthy, efficient, and cost-saving green buildings. It aims to improve building and construction project performance across seven areas of environmental and human health.
-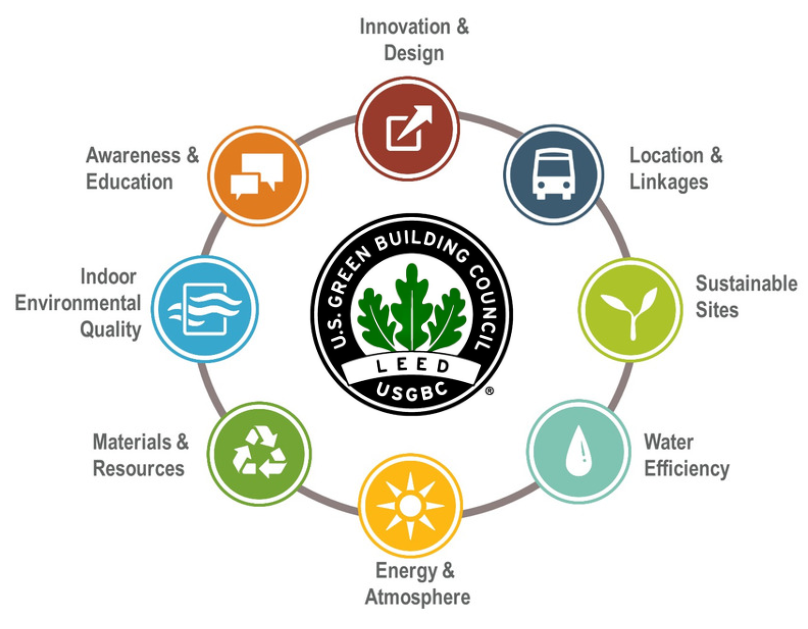
-
-To achieve LEED certification, a project earns points by adhering to prerequisites and credits that address carbon, energy, water, waste, transportation, materials, health and indoor environmental quality. Projects go through a verification and review process by GBCI and are awarded points that correspond to a level of LEED certification: **Certified (40-49 points), Silver (50-59 points), Gold (60-79 points) and Platinum (80+ points)**.
-
\ No newline at end of file
diff --git a/Software_Carbon_Efficiency_Rating(SCER)/SCER for Database Server Software.md b/Software_Carbon_Efficiency_Rating(SCER)/SCER for Database Server Software.md
deleted file mode 100644
index 70d6846..0000000
--- a/Software_Carbon_Efficiency_Rating(SCER)/SCER for Database Server Software.md
+++ /dev/null
@@ -1,186 +0,0 @@
-# SCER Specification for Relational Database Server Software
-
-## Version 0.1
-
-### Abstract
-
-The Software Carbon Efficiency Rating (SCER) for Relational Database Server Software provides a framework for assessing the carbon efficiency of database management systems (DBMS) based on energy consumption and operational efficiency.
-
-### Table of Contents
-
-1. [Introduction](#1-introduction)
-2. [Objective](#2-objective)
-3. [Terminology](#3-terminology)
-4. [Scope](#4-scope)
-5. [Software Categorization](#5-software-categorization)
-6. [Benchmarking](#6-benchmarking)
-7. [Rating System](#7-rating-system)
-8. [Rating Calculation Algorithm](#8-rating-calculation-algorithm)
-9. [Compliance and Verification](#9-compliance-and-verification)
-10. [Future Directions](#10-future-directions)
-
----
-
-### 1. Introduction
-
-Database servers are integral to IT infrastructure with substantial energy footprints. Enhancing their carbon efficiency is vital for minimizing the environmental impact of data-centric operations worldwide.
-
-### 2. Objective
-
-To define and implement a standard that measures and rates the carbon efficiency of relational database server software, encouraging industry progression towards more sustainable practices.
-
-### 3. Terminology
-
-- **SCER**: Software Carbon Efficiency Rating
-- **DBMS**: Database Management System
-- **OPS/Watt-hour**: Operations Per Second per Watt
-- **Carbon Footprint**: The total CO2e emissions associated with the DBMS across its lifecycle.
-
-### 4. Scope
-
-This standard applies to relational database server software encompassing open-source and proprietary systems.
-
-### 5. Software Categorization
-
-Database server software is categorized based on:
-
-- Scale: small, medium, large
-- Use case: transactional, analytical, hybrid
-- Deployment model: on-premises, cloud-based, hybrid
-
-### 6. Benchmarking
-
-Benchmarking focuses on:
-
-- **OPS/Watt-hour**: Database operations executed per watt hour of power consumed.
-- **Transaction Efficiency**: Energy consumed per completed transaction.
-- **Query Optimization**: Energy cost of executing complex queries.
-
-### 7. Rating System
-
-SCER classifications:
-
-- **A**: Highly efficient operations (>X OPS/Watt-hour), advanced query optimization, low energy per transaction.
-- **B**: Moderately efficient ([Y-X] OPS/Watt-hour), standard query optimization, average energy per transaction.
-- **C**: Less efficient (X Streams/Watt-hour), high data transfer efficiency, and optimal user engagement.
-- **B**: Moderately efficient ([Y-X] Streams/Watt-hour), moderate data transfer efficiency, and user engagement.
-- **C**: Less efficient (
- **N**: Not similar
-
-- Relational Database Server Category Example:
-
- | Software Applications| Purpose and Functionality | Platform and Deployment |End User Base|
- | -------- | :---------:| :---------:|:---------:|
- | MiSQL | S | S |S |
- | PSQL | S | S |S |
- | SQLite | S | **N** |S |
-
- In this example, SQLite is a light weight database which is mostly used and deployed in mobile phone platforms that are significantly different from MiSQL and PostgresSQL, so SQLite should not belong in this category, and should not be used for SCER rating in this category.
-
-##### 2.1.2 Extended Components of a Software Categorization
-
-The following aspects may be extended in determining if the software application are in the same category:
-
-- **Type of License:** Differentiating software based on open-source or proprietary status and licensing models.
- - **Open Source vs. Proprietary:** Linux kernel as open-source software vs. Microsoft Windows as proprietary software.
- - **Licensing Model:** Subscription-based model like Adobe Creative Cloud versus a one-time purchase software like Final Cut Pro.
- - **Example Categories:**
- - **Open Source:** Mozilla Firefox: Open-source web browser. LibreOffice: Open-source office suite.
- - **Proprietary:** Microsoft Office: Proprietary office suite. Adobe Acrobat: Proprietary PDF solution.
-
-- **Technical Complexity:** Evaluating the software's architecture and dependencies.
- - **Architecture:** Docker containers showcasing microservices architecture.
- - **Dependencies:** Node.js applications often depend on numerous packages from npm (node package manager).
- - **Example Categories:**
- - **Data Processing:** Hadoop: Framework for distributed storage and processing of large data sets. Elasticsearch: Search and analytics engine for all types of data.
- - **Content Management Systems:** Joomla: Content management system for web content. Drupal: Content management system for complex websites.
-
-- **Integration and Ecosystem:** Assessing compatibility and ecosystem integration.
- - **Compatibility:** Slack integrates with numerous other productivity tools like Trello, Asana, and Google Drive.
- - **Ecosystem:** Apple’s iOS apps that are part of the broader Apple ecosystem, designed to work seamlessly with other Apple devices and services.
- - **Example Categories:**
- - **Customer Relationship Management (CRM):** Salesforce: CRM solution with extensive integration capabilities. HubSpot CRM: Inbound marketing, sales, and service software with integration features.
- - **Development Tools:** JetBrains IntelliJ IDEA: Integrated development environment for software development. Microsoft Visual Studio: Comprehensive development environment with extensive integrations.
-
-- **Regulatory and Compliance Considerations:** Ensuring compliance with applicable regulations and standards.
- - **Healthcare Software:** HIPAA-compliant patient management systems like Cerner.
- - **Financial Software:** SEC-compliant trading platforms like TD Ameritrade.
- - **Example Categories:**
- - **Financial Services:** Thomson Reuters Eikon: Financial data and analytics tool. FactSet: Financial data and software for investment professionals.
- - **Telecommunications:** Amdocs: Software and services for communications, media, and financial services providers. Ericsson: Network software for telecom operators.
-
-- **Feedback and Market Recognition:** Using market recognition and user feedback for category validation.
- - **User Reviews:** Yelp for restaurant and business reviews.
- - **Awards and Recognition:** Autodesk AutoCAD receiving awards for its CAD design software capabilities.
- - **Example Categories:**
- - **Web Browsers:** Google Chrome: Highly rated for speed and integration with Google services. Mozilla Firefox: Praised for privacy features and open-source development.
- - **E-Commerce Platforms:** Shopify: Widely recognized platform for creating online stores. Magento: Renowned open-source e-commerce platform.
-
-- **Industry Vertical:** Classifying software according to the relevant industry vertical.
- - **Healthcare:** Epic Systems for electronic health records management.
- - **Finance:** QuickBooks for accounting in small to mid-sized businesses.
- - **Example Categories:**
- - **Healthcare:** McKesson: Medical supplies ordering and healthcare information technology. Allscripts: Provider of electronic record, practice management, and other clinical solutions.
- - **Education:** Blackboard: Learning management system for education providers. Canvas: Web-based learning management system for educational institutions.
-
-- **Consistent Review and Update:**
- - **Periodic Review:** Google Chrome, which releases updates frequently to reflect the latest web standards and security practices.
- - **Benchmark against Peers:** Comparing Microsoft Office 365 to other productivity suites like Google Workspace for feature set and market position.
- - **Example Categories:**
- - **Operating Systems:** Windows 10: Regular feature updates and security patches. macOS: Annual updates with feature enhancements and security improvements.
- - **Security Software:** Symantec Norton: Consistent updates for virus definitions and security features. McAfee: Frequent updates to maintain security and performance standards.
-
-- **Size of the Software:** Many softwares are comparatively very big in size and this may get multiplied with each install by different users.
- - **Software Size itself:** Software size and depending on the type of installation required for the application, it's size can have an huge impact on the carbon emissions generated by the software.
- - **Dependencies:** Node.js or Angular applications depend on numerous packages and that also needs to be downloaded with each installation.
- - **Examples:**
- - **Desktop/Mobile Applications:** Microsoft Office 365, Visual Studio, OS, etc.
- - **Web Applications:** Microsoft 365 Apps, Open Cloud Architecture, Virtual work environment, etc.
-
-Note:
-1. Should SaaS (Software s a Service) be part of the software categorization? It most likely is part of the software categorization for the SCER purpose, such as music streaming service. It seems that SCER really means software *induced* carbon efficiency rating - meaning, the carbon efficiencies due to running of the software application, regardless if the software is run as a service, or run on a desktop.
-2. Should these aspects of categorizing software be OR or AND relationship? Does all software applications belonging to the same category have to have the same answer for each aspect? For example, should microsoft office and libre office belong to the same office suite software category for the purpose of SCER rating, even though one is proprietary, the other is open source?
-3. Are these too heavy? Any other considerations missing?
-
----
-
-#### 2.2 Benchmarking Specification
-
-This section of the SCER Specification provides a detailed description of the benchmarking process for measuring the carbon efficiency of software applications. It includes the selection of appropriate metrics, the definition of the benchmarking workload, the necessary test environment setup, and the methodology for executing the benchmarks.
-
-##### 2.2.1 Definition of Benchmarks
-
-Benchmarks serve as the comparative performance standards against which software applications are evaluated for their [Software Carbon Intensity (SCI)](https://github.com/Green-Software-Foundation/sci/blob/main/Software_Carbon_Intensity/Software_Carbon_Intensity_Specification.md) scores or [Social Cost of Carbon (SCC)](https://www.brookings.edu/articles/what-is-the-social-cost-of-carbon/#:~:text=The%20social%20cost%20of%20carbon%20(SCC)%20is%20an%20estimate%20of,a%20ton%20of%20carbon%20emissions.) dollars. The default measurement of benchmarks is in SCI scores.
-
-##### 2.2.2 Unit of Software Function (USF)
-
-The "Unit of Software Function" or USF refers to the most commonly used function of the software, such as:
-
-- **Read/Write Operations** for database software
-- **Rendering a Frame** for graphical software or video games
-- **Handling a Web Request** for web servers
-- **Processing a Data Block** for data processing software
-
-##### 2.2.3 Standardized Tests or Benchmarking Workload
-
-For each category of software, a standardized test can be developed to measure the functional utility of the software, which is then used to calculate its SCI score. The tests will simulate the most commonly used function of the software.
-
-The workload used for benchmarking should be representative of real-world scenarios to ensure the results are relevant and actionable.
-
-- **Workload Profiles**: Detailed descriptions of the different types of workload the software will be subjected to during the benchmarking process.
-- **Workload Metrics**: The quantitative measures of each workload profile, such as the number of simultaneous users, data size, and operation mix.
-
-Example Workload Profiles:
-- For a video streaming service, the workload might simulate streaming at various resolutions.
-- For a customer relationship management (CRM) system, the workload could involve a mix of database reads/writes, report generations, and user queries.
-
-##### 2.2.4 SCI Score Formula
-
-The SCI Score for a software application will be calculated as follows:
-
-$$\ SCI\ Score = \frac{CO2e\ emissions}{USF} \$$
-
-Where CO2e emissions are measured in kilograms and USF is determined by the benchmark test.
-
-Default measurement is in SCI but other metrics can be used to base the SCER rating, such as kWh, SCC, SCI, CO2e, etc.
-
-##### 2.2.5 Test Environment
-
-The test environment must be standardized to minimize variability in the benchmark results.
-
-- **Hardware Specifications**: A description of the physical or virtual hardware on which the software will be tested, including processor, memory, storage, and networking details.
-- **Software Configuration**: The operating system, middleware, and any other software stack components should be specified and standardized.
-
-Example Test Environments:
-- A specified cloud instance type with a defined operating system and resource allocation.
-- A physical server with controlled ambient temperature and power supply to ensure consistent results.
-
-##### 2.2.6 Test Methodology
-
-A detailed, repeatable method for conducting the benchmarks.
-
-- **Setup Instructions**: Step-by-step guidance to prepare the test environment, including installation and configuration of the software and tools.
-- **Execution Steps**: A sequential list of actions to perform the benchmark, including starting the software, initiating the workload, monitoring performance, and recording results.
-- **Result Collection and Analysis**: Guidelines for gathering the data, ensuring its integrity, and analyzing it to derive meaningful insights.
-
-Example Test Methodology:
-- For a web application, steps might include setting up a load generator, executing predetermined tasks, and measuring response times and energy consumption.
-- For a data processing application, it might involve running specific data sets through the software and measuring the time and energy required for processing.
-
-Each of these components should be defined with sufficient detail to enable consistent replication of the benchmarking process across different environments and software versions. This standardization is crucial for ensuring that the SCER ratings are reliable and comparable across different software applications.
-
-In summary, the following table can serve as a template or checklist when defining benchmarks:
-
-| Benchmark Measurement| Benchmark Workload | Test Environment |Test Methodology|
-| -------- | ---------| ---------|---------|
-| kWhSCCSCI (default) CO2e, or Other | Unit of Software Function (USF) Number of times to execute USF | Hardware specificationsSoftware Configuration |Setup InstructionsExecution StepsResult Collection and Analysis |
-
----
-*Benchmark measurement shall be carbon related, by default is in SCI scores. However, if the benchmarking is done in a controlled environment, all other variables related to carbon emission are being equal, then benchmarking can be measured in energy consumed (e.g. kWh) rather than in SCI.*
-
-#### 2.3 Rating Specification
-##### 2.3.1 **Rating Components:**
-The common components of a rating specification includes the following:
-1. Rating Scale: Define the rating scale and the criteria for each rating level.
-2. Evaluation Methodology: Detail the evaluation methodology for converting benchmark results to ratings.
-3. Reporting and Disclosure: Standardized format for rating disclosure.
-
-##### 2.3.2 **Rating Algorithm:**
-To compute the SCER (Software Carbon Efficiency Rating) based on SCI Score performance in relation to peer software within the same category.
-
-1. **Data Collection**: Gather SCI Scores from multiple software applications within the same category.
-2. **Normalization**: Normalize SCI Scores to create a common scale.
-3. **Ranking**: Rank software based on normalized SCI Scores.
-4. **Percentile Calculation**: Calculate the percentile position for each software application:
-
-$$\ Percentile\ Position = \left( \frac{Rank - 1}{Total\ Number\ of\ Submissions\ -\ 1} \right) \times 100 \$$
-
-5. **Rating Assignment**: Based on the percentile position, assign a rating according to the pre-defined scale. A default rating is A to C.
-
-##### 2.3.3 **Rating Example**
-Here is a SCER rating example, where the SCI scores of 5 software applications in the same category are collected. Lower SCI (Software Carbon Intensity) scores indicate higher software carbon efficiency. The rating scale includes labels A through C:
-
-- **A**: Exceptionally efficient (percentile position above the 90th percentile).
-- **B**: Above average efficiency (percentile position from the 60th to 90th percentile).
-- **C**: Average to inefficient (percentile position below the 60th percentile).
-
-**Collected SCI Scores:**
-
-- Software 1: SCI Score of 100
-- Software 2: SCI Score of 150
-- Software 3: SCI Score of 160
-- Software 4: SCI Score of 200
-- Software 5: SCI Score of 250
-
-**Rating Assignment Process:**
-
-###### Step 1: Data Collection
-
-SCI Scores for five software applications have been collected.
-
-###### Step 2: Ranking
-
-The software applications are ranked by SCI Score, from highest to lowest:
-
-1. Software 5: 250
-2. Software 4: 200
-3. Software 3: 160
-2. Software 2: 150
-1. Software 1: 100
-
-###### Step 3: Percentile Calculation
-
-Percentile positions are calculated using the formula:
-
-$$\ Percentile\ Position = \left( \frac{Rank - 1}{Total\ Number\ of\ Submissions\ -\ 1} \right) \times 100 \$$
-
-- Software 5: 0%
-- Software 4: 25%
-- Software 3: 50%
-- Software 2: 75%
-- Software 1: 100%
-
-###### Step 4: Rating Assignment
-
-Using the rating scale:
-
-- **A**: Above 90%
-- **B**: 60% to 90%
-- **C**: Below 60%
-
-###### Final Ratings by Efficiency
-
-- Software 1: **A**
-- Software 2: **B**
-- Software 3: **C**
-- Software 4: **C**
-- Software 5: **C**
-
-
-The final ratings reflect the carbon efficiency of the software applications, with Software 3-5 being average to inefficient, Software 2 above average, and Software 1 being exceptionally efficient.
-
-In summary, the following table can serve as a template or checklist when rating:
-
-| Rating Scale | Rating Algorithm | Reporting and Disclosure |
-| -------- | ---------| ---------|
-| e.g. A - C, or A, A+, A++, or AAA, etc. Default is A (>90%), B (60-89%),C (< 60%) |e.g. getting data, calculate mean, average etc. | e.g. rating assignment and reporting |
----
-
-
-#### 2.4 SCER Rating Visualization and Labeling
-
-
-
-
-
-### 3. Creating Category-Specific SCER Specifications
-
-This section describes guidelines for adapting the SCER Specification to specific software categories.
-
-Different industries may require different ways of benchmarking and rating system for software. Therefore, this specification is defined with flexibility in mind so that it can be adapted for different industry use cases. This means that **this SCER specification is a specification of specification, or a meta-specification.** Category-specific specification can be derived from this base specification. A separate document describes an example of how to use the base specification to create a category specific specification, and examples were given on how to create the category specific specification.
-
----
-
-### 4. Appendices
-
-Supporting documents, example calculations, and reporting templates.
-
----
-
-### 5. References
-
-List of references used in the creation of the SCER Specification.
-
----
-
diff --git a/Software_Carbon_Efficiency_Rating(SCER)/license.md b/Software_Carbon_Efficiency_Rating(SCER)/license.md
deleted file mode 100644
index 5043f09..0000000
--- a/Software_Carbon_Efficiency_Rating(SCER)/license.md
+++ /dev/null
@@ -1,15 +0,0 @@
-Materials in this repository other than source code are provided as follows:
-
-Copyright (c) 2021 Joint Development Foundation Projects, LLC, GSF Series and its contributors. All rights reserved. THESE MATERIALS ARE PROVIDED "AS IS." The parties expressly disclaim any warranties (express, implied, or otherwise), including implied warranties of merchantability, non-infringement, fitness for a particular purpose, or title, related to the materials. The entire risk as to implementing or otherwise using the materials is assumed by the implementer and user. IN NO EVENT WILL THE PARTIES BE LIABLE TO ANY OTHER PARTY FOR LOST PROFITS OR ANY FORM OF INDIRECT, SPECIAL, INCIDENTAL, OR CONSEQUENTIAL DAMAGES OF ANY CHARACTER FROM ANY CAUSES OF ACTION OF ANY KIND WITH RESPECT TO THIS DELIVERABLE OR ITS GOVERNING AGREEMENT, WHETHER BASED ON BREACH OF CONTRACT, TORT (INCLUDING NEGLIGENCE), OR OTHERWISE, AND WHETHER OR NOT THE OTHER MEMBER HAS BEEN ADVISED OF THE POSSIBILITY OF SUCH DAMAGE.
-
-The patent mode selected for materials developed by this Working Group is W3C Mode. For specific details, see this Working Group's Charter at:
-
-Source code in this repository is provided under the MIT License, as follows:
-
-Copyright (c) 2021 Joint Development Foundation Projects, LLC, GSF Series and its contributors.
-
-Permission is hereby granted, free of charge, to any person obtaining a copy of this software and associated documentation files (the "Software"), to deal in the Software without restriction, including without limitation the rights to use, copy, modify, merge, publish, distribute, sublicense, and/or sell copies of the Software, and to permit persons to whom the Software is furnished to do so, subject to the following conditions:
-
-The above copyright notice and this permission notice shall be included in all copies or substantial portions of the Software.
-
-THE SOFTWARE IS PROVIDED "AS IS", WITHOUT WARRANTY OF ANY KIND, EXPRESS OR IMPLIED, INCLUDING BUT NOT LIMITED TO THE WARRANTIES OF MERCHANTABILITY, FITNESS FOR A PARTICULAR PURPOSE AND NONINFRINGEMENT. IN NO EVENT SHALL THE AUTHORS OR COPYRIGHT HOLDERS BE LIABLE FOR ANY CLAIM, DAMAGES OR OTHER LIABILITY, WHETHER IN AN ACTION OF CONTRACT, TORT OR OTHERWISE, ARISING FROM, OUT OF OR IN CONNECTION WITH THE SOFTWARE OR THE USE OR OTHER DEALINGS IN THE SOFTWARE.
diff --git a/use_cases/SCER_FOR_LLM/SCER_FOR_LLM_Work_Plan.md b/use_cases/SCER_FOR_LLM/SCER_FOR_LLM_Work_Plan.md
deleted file mode 100644
index fa7a915..0000000
--- a/use_cases/SCER_FOR_LLM/SCER_FOR_LLM_Work_Plan.md
+++ /dev/null
@@ -1,159 +0,0 @@
-# SCER for LLM Work Plan
-
-## Background and Motivation
-
-Now that the SCER specification is fully developed, there's an apparent gap in its application, primarily due to the lack of use cases that demonstrate its utility. The recent rapid AI development reveals a critical need in the Generative AI (GAI) domain: a standardized method for evaluating and comparing the carbon efficiency of Large Language Models (LLMs). Therefore, the aim of SCER for LLM is to establish a framework that provides a relative or absolute rating of LLMs carbon efficiencies.
-
-## Landscape Research and Analysis
-
-### Energy Consumption by LLMs
-
-Recent advancements and policy mandates on AI's energy needs underscore the importance of sustainable practices in AI development. An in-depth analysis of the current energy consumption trends by LLMs is essential. For a comprehensive overview, refer to the study at [NNLabs on Power Requirements of Large Language Models](https://www.nnlabs.org/power-requirements-of-large-language-models/).
-
-[](https://www.cbinsights.com/research/report/generative-ai-predictions-2024/)
-
-Source: [CBInsight GenAI Predictions for 2024](https://www.cbinsights.com/research/report/generative-ai-predictions-2024/)
-
-### Energy Consumption vs. Carbon Footprint:
- - **Energy Consumption**:
- - Measures the electrical power LLMs require, in kilowatt-hours.
- - Focuses on direct energy use by hardware for operations like training and inference.
- - **Carbon Footprint**:
- - Accounts for CO2 and other greenhouse gases emitted due to LLMs' energy use, measured in CO2e.
- - Considers both direct and indirect emissions, including the energy source's environmental impact.
-
-- **Key Differences**:
- - Energy consumption is a direct measure of electricity used, not reflecting the energy source's carbon intensity.
- - The carbon footprint offers a comprehensive view of environmental impact, including the emissions from producing the consumed energy.
-
-- **Relationship**:
- - While related, they're not equivalent; the carbon footprint also accounts for the type of energy source, making it possible for high energy consumption to have a low carbon footprint if renewable energy sources are used.
-
-When rating the carbon efficiencies of LLMs, CO2e (carbon dioxide equivalent) may be the preferred unit over kWh (kilowatt-hour) because:
-
-- **CO2e** measures the comprehensive environmental impact, including all greenhouse gas emissions related to energy consumption of LLMs. It accounts for the carbon intensity of different energy sources, offering a holistic view of an LLM's carbon footprint.
-
-- **kWh** only reflects energy consumption without indicating the environmental impact or carbon intensity of the energy source.
-### If the underlying CO2e measuring test bed stays the same, is CO2e still prefered than kWh?
-Using CO2e enables meaningful comparisons and insights into the sustainability and environmental friendliness of LLM technologies, aligning with global sustainability objectives.
-Even with a standardized CO2e test bed, using CO2e over kWh for rating LLMs is preferred due to:
-
-- **Consistent Environmental Impact Measurement**: Ensures comparability and reflects the true environmental cost.
-- **Sustainability Goals Alignment**: Emphasizes reducing greenhouse gas emissions, promoting environmentally friendly AI technologies.
-- **Comprehensive Emissions Accounting**: Captures all greenhouse gases, offering a more accurate assessment of climate impact.
-- **Renewable Energy Incentive**: Encourages the use of cleaner energy sources by showcasing lower CO2e ratings for LLMs powered by renewable energy.
-
-This approach focuses on environmental sustainability and the broader impact of LLMs beyond mere energy consumption.
-
-### Separating the carbon efficiency ratings of LLMs into training and inference phases
-
-1. **Different Energy Profiles**: Training is more energy-intensive than inference, necessitating distinct evaluations.
-2. **Lifecycle Insights**: Offers clear insights into where interventions can most effectively reduce the carbon footprint.
-3. **Operational Efficiency Optimization**: Enables identification of optimization opportunities for both training and inference.
-4. **Transparency and Accountability**: Enhances understanding of the environmental impact of developing and using LLMs.
-5. **Encourages Sustainable Practices**: Motivates the development of energy-efficient algorithms and greener AI lifecycle practices.
-
-Assessing training and inference separately improves the accuracy of carbon efficiency ratings and supports minimizing the environmental impact of AI technologies.
-
-### GenAI Life-cycle Environmental Impact
-Here is a great article from [LinkedIn Post on MPG for LLMs](https://www.linkedin.com/pulse/mpg-llms-exploring-energy-efficiency-generative-ai-gamazaychikov/). Here are some brief excerpts:
-
-
-
-
-
-
-
-
-
-
-### Food for thoughts:
-- **Please note that the LLM's measurements from Huggingface and ML.Energy are measured in Energy Consumption units(tokens/kwh/joules), not CO2e.**
-
-- **According to "GenAI Life-cycle Environmental Impact", measuring CO2e for LLMs may involve too much more to account for CO2e measurement?**
-
-- **Does it mean that in principle, the measurement should be done in CO2e, but in reality, kWh is more practical?**
-
-### A Case Study: Hugging Face's Initiatives on LLM's Carbon Footprint
-
-Hugging Face, an AI startup, has taken notable steps toward understanding and mitigating the environmental impact of large language models (LLMs). These models, while powerful, have a hidden cost: their substantial energy consumption during training and operation. Here are more details on some of Hugging Face's initiatives:
-
-1. **Estimating Broader Carbon Footprint**:
- - Hugging Face's pioneering involves estimating the **whole life cycle emissions** of LLMs, not just those during training.
- - They calculated emissions for their own LLM, [**BLOOM**](https://huggingface.co/bigscience/bloom), which was launched recently.
- - The process involved considering various factors:
- - Energy used for model training on a supercomputer.
- - Manufacturing energy for the supercomputer's hardware.
- - Energy needed to maintain computing infrastructure.
- - Energy consumed during BLOOM's runtime.
- - By using the [**CodeCarbon**](https://codecarbon.io/) software tool, they tracked BLOOM's real-time carbon dioxide emissions over 18 days.
- - The result: BLOOM's training led to **25 metric tons** of carbon dioxide emissions.
- - However, when accounting for manufacturing, infrastructure, and operational energy, this figure doubled to **50 metric tons**.
- - Remarkably, BLOOM's emissions are lower than other LLMs of similar size due to being trained on a French supercomputer powered mostly by **nuclear energy**, which emits no CO₂. In contrast, models trained in regions relying on fossil fuels may be more polluting.
-
-2. **Daily Emissions**:
- - After BLOOM's launch, Hugging Face estimated that using the model emitted around **19 kilograms** of carbon dioxide per day.
- - To put this in perspective, it's akin to the emissions produced by driving approximately **54 miles** in an average new car.
- - By comparison, OpenAI's **GPT-3** and Meta's **OPT** emitted over **500** and **75 metric tons** of CO₂ during training, respectively.
- - GPT-3's higher emissions partly stem from being trained on older, less efficient hardware.
-
-3. **Setting a New Standard**:
- - Hugging Face's work sets a precedent for organizations developing AI models.
- - It provides much-needed clarity on LLMs' carbon footprints.
- - As we continue to explore AI's environmental impact, initiatives like these are crucial for responsible development and deployment.
-
-Hugging Face's efforts shed light on the carbon footprint of LLMs, emphasizing the need for sustainable practices in AI development.
-
-
-### Carbon Footprint Estimation Tools for LLMs
-A critical examination of the existing tools designed to measure the carbon footprint of LLMs will help reveal their strengths and weaknesses. A gap analysis will help identify the unmet needs in the current ecosystem.
-
-**CodeCarbon** is an open-source software tool designed to estimate the carbon footprint associated with the computing power used for training AI models, including Large Language Models (LLMs). It tracks the energy consumption of computing resources and estimates CO2 emissions based on the energy mix of the location where the computation occurs. CodeCarbon aims to raise awareness about the environmental impact of computational tasks and encourage more sustainable practices in AI research and development.
-
-**Equivalents and Similar Tools**:
-
-1. [**ML CO2 Impact**](https://mlco2.github.io/impact/): A tool that calculates the carbon impact of machine learning models by considering the energy consumption and the specific energy grid's carbon intensity.
-
-2. [**Green Algorithms**](https://www.green-algorithms.org): Offers a way to estimate the carbon footprint of computational tasks, providing insights into the environmental impact of research computations.
-
-3. [**Carbontracker**](https://github.com/lfwa/carbontracker): This tool monitors and predicts the energy usage and carbon footprint of training deep learning models, allowing researchers to understand and reduce their models' environmental impact.
-
-**Tools Comparison**
-
-| Tool | Pros | Cons |
-|-----------------|----------------------------------------------------------|-----------------------------------------------------------|
-| **CodeCarbon** | - User-friendly and easy integration.
- Supports multiple environments.
- Provides actionable insights. | - Depends on accurate energy mix data.
- Limited real-time energy source tracking. |
-| **ML CO2 Impact** | - Simplifies carbon emissions calculation for ML projects.
- Raises environmental impact awareness. | - Requires manual input of energy data.
- Lacks real-time monitoring. |
-| **Green Algorithms** | - Broad estimation capabilities beyond ML.
- Offers offsetting recommendations. | - Overly simplistic estimations for complex tasks.
- Might not capture computing nuances. |
-| **Carbontracker** | - Real-time energy and carbon footprint tracking.
- Useful for comparing model efficiencies. | - Best suited for deep learning, not all computational tasks.
- Integration requires more effort. |
-
-
-## SCER for LLM Overview
-
-SCER for LLM proposes a standardized approach to measure and rate the carbon efficiency of LLMs. This initiative is positioned to bridge the identified gaps by providing a clear specification for evaluating LLMs' environmental impact. SCER aims to facilitate the adoption of more sustainable practices in the development and deployment of generative AI technologies.
-### The case for SCER for LLM
-
-**The Question**: Why carbon efficiency ratings are not part of the evaluation parameter in the most popular LLMs in Hugging Face? Is it because Carbon Efficiencies of LLMs are still not a priority in evaluating LLMs for deployment/applications?
-
-[](https://huggingface.co)
-
-## Goal and Vision of SCER for LLM
-
-The primary goal of SCER for LLM is to promote transparency and accountability in the AI sector by standardizing the measurement of LLMs' carbon efficiencies. The vision encompasses a future where all stakeholders in the AI ecosystem, from developers to end-users, are empowered to make informed decisions based on the carbon efficiency ratings of LLMs. Ultimately, SCER for LLM seeks to encourage the development of more carbon-efficient AI technologies, contributing to the global efforts to fight climate change.
-
-
-## Action Items
-
-
-1. **Research and Analysis**:
- - Conduct a comprehensive review of the latest development regarding AI carbon footprint trend and concerns
- - Perform a gap analysis on existing carbon measurement tools, highlighting areas for improvement or innovation.
- - Research on Hugging Face's carbon efficiency evaluation process for LLMs, and to identify potential gaps and opportunities where SCER for LLM can provide value.
-
-3. **Stakeholder Engagement**: Engage with key stakeholders across the AI ecosystem, including technology developers, regulatory bodies, and end-users, to gather insights and foster collaboration.
-
-4. **Standard Development**: Based on the gap analysis, develop or enhance tools that accurately measure the carbon efficiencies of LLMs in various operational scenarios. This includes the software categorization, benchmark definition, rating method, algorithm, and visualization and labelling.
-
-5. **Pilot Testing**: Implement pilot projects to test the efficacy of SCER ratings for LLMs in real-world applications, collecting data to refine and validate the SCER framework.
-
-6. **Outreach and Education**: Launch initiatives aimed at raising awareness about the importance of carbon efficiency in LLMs, targeting both the AI community and the broader public.
diff --git a/use_cases/SCER_FOR_LLM/SCER_For_LLM_Specification.md b/use_cases/SCER_FOR_LLM/SCER_For_LLM_Specification.md
deleted file mode 100644
index a7e94e2..0000000
--- a/use_cases/SCER_FOR_LLM/SCER_For_LLM_Specification.md
+++ /dev/null
@@ -1,154 +0,0 @@
-## SCER Specification for Rating Carbon Efficiencies of LLMs
-
-**Motivation and Value Statement for the SCER Specification for LLMs:**
-
-The SCER (Software Carbon Efficiency Rating) specification, pronounced “sheer”, provides a crucial framework designed to standardize the assessment and comparison of software applications based on their carbon efficiencies. Without an industry-standard approach to measure the environmental impact of software, there is currently no systematic method to assess or compare the "greenness" of software relative to similar applications within the same category. By adopting this specification, software "greenness" is established and recognized as a key performance indicator (KPI) in the evaluation of software solutions. This not only promotes a focus on sustainability but also advances the broader agenda of sustainable software development, aligning with global environmental goals.
-
-The SCER Specification for Rating Carbon Efficiencies of LLMs serves as a practical application for [the foundational SCER specification](https://github.com/Green-Software-Foundation/scer/blob/Dev/Software_Carbon_Efficiency_Rating/Software_Carbon_Efficiency_Rating_Specification.md). Following the standard process outlined in the SCER specification, the components detailed below are designed to assess the carbon efficiencies of LLMs:
-1. Software Categorization (model size, types, etc)
-1. Benchmark definition (workload, method, tools/infra, etc)
-1. Rating definition (range, algorithm, etc)
-1. Visualization and Labeling (visuals, placement, access to sources/details, etc)
-
----
-Below, each component of the specification is defined in detail, using Hugging Face as a case study or example.
-
-### 1. Software Categorization
-- **Sub-categories for LLMs**
- - Define categories based on:
- - Model size (e.g., small, medium, large, extra-large)
- - Application type (e.g. text generation, translation, summarization)
- - In [Hugging Face Open LLM Leaderboard](https://huggingface.co/spaces/HuggingFaceH4/open_llm_leaderboard), more sub-categories/filters are defined:
- - Model types (pretrained, continuously pretrained, fine-tuned on domain-specific datasets)
- - Precision (float16, bfloat16, 8 bit, 4 bit, GPTQ, etc)
- - Model sizes (1.5 to 70+ billions of parameters)
-
- *Note*: In Generative AI, application types can include text (via LLMs), pictures, videos (such as [Sora by OpenAI](https://openai.com/sora)), music/sound (including Meta's AudioCraft, Google's MusicFX, and [Suno](https://suno.com/)), speech, and data synthesis, etc. While the current specification is tailored to LLMs, future versions may expand to cover other Generative AI application types. Nonetheless, the general methodology described herein is applicable across all types of applications.
-
-### 2. Benchmark Definition
-- **Standard Workloads**
- - Identify common tasks for LLMs that reflect real-world usage, like text completion, language translation, and fine-tuning performance.
- - In Hugging Face, a set of [6 benchmarks or workloads](https://huggingface.co/datasets/open-llm-leaderboard/results) (mostly as open source projects or from research papers) are run against the submitted LLMs:
- 1. AI2 Reasoning Challenge (ARC) - Grade-School Science Questions (25-shot)
- 1. HellaSwag - Commonsense Inference (10-shot)
- 1. MMLU - Massive Multi-Task Language Understanding, knowledge on 57 domains (5-shot)
- 1. TruthfulQA - Propensity to Produce Falsehoods (0-shot)
- 1. Winogrande - Adversarial Winograd Schema Challenge (5-shot)
- 1. GSM8k - Grade School Math Word Problems Solving Complex Mathematical Reasoning (5-shot)
-
-- **Measurement Methods**
- - Develop methodologies for measuring carbon efficiency, including real-time monitoring or simulating typical deployment scenarios.
- - Hugging Face uses [CodeCarbon](https://mlco2.github.io/codecarbon/) to measure energy consumption. CodeCarbon takes into consideration of the GPU, CPU, RAM and location of the machine. Hugging Face uses kWh in evaluating the energy efficiencies of LLMs:
- [](https://huggingface.co/spaces/optimum/llm-perf-leaderboard)
- - CodeCarbon does provide CO2e information, in addition to kWh:[](https://codecarbon.io/)
-- **Tooling and Infrastructure**
- - Specify required tools or platforms for conducting benchmarks to ensure *reproducibility* and transparency in the testing process.
- - From Hugging Face [LLM-Perf Leaderboard](https://huggingface.co/spaces/optimum/llm-perf-leaderboard):
- - Hardware: [H100 80GB 350W](https://www.amazon.com/NVIDIA-Graphic-Memory-900-21010-0000-000-Warraty/dp/B0C957FN64?source=ps-sl-shoppingads-lpcontext&ref_=fplfs&psc=1&smid=A3LRS18WTQPMZ4), [A100 80GB 275W](https://www.cdw.com/product/pny-nvidia-a100-80gb-pcie-gen-4-graphic-card/7065275), and [RTX4090-24GB-450W](https://www.bestbuy.com/site/nvidia-geforce-rtx-4090-24gb-gddr6x-graphics-card-titanium-black/6521430.p?skuId=6521430) are the GPU hardware used in the benchmark infrastructure
- - Software:
- - [optimum-benchmark](https://github.com/huggingface/optimum-benchmark): A unified multi-backend utility for benchmarking Transformers, Timm, Diffusers and Sentence-Transformers with full support of Optimum's hardware optimizations & quantization schemes.
- - [Eleuther AI Language Model Evaluation Harness](https://github.com/EleutherAI/lm-evaluation-harness), a unified framework to test generative language models on a large number of different evaluation tasks, is used to evaluate LLMs on 6 key benchmarks.
-
-
-
-### 3. Rating Definition
-- **Efficiency Range**
- - Determine what constitutes low, medium, and high efficiency based on carbon output per unit of computational output or per unit of software function (USF), e.g. carbon per 1,000 tokens/words generated.
- - *LLM Performance Efficiency*: Hugging Face uses the average score of 6 performance benchmark results, in the range of 0 to 100:
- [](https://huggingface.co/spaces/HuggingFaceH4/open_llm_leaderboard)
- - *LLM Energy Efficiency*: measured in ''tokens/kWh''. Hugging Face uses CodeCarbon to get energy consumed number in kWh, which is then divided by the number of tokens generated, as shown here:
- [](https://huggingface.co/spaces/optimum/llm-perf-leaderboard)
- One observation is that there appears to be a trade-off between energy efficiency and performance in LLMs; as energy efficiency increases, average performance tends to decrease. This pattern is understandable given that larger language models typically outperform smaller ones but at the expense of higher energy consumption, as demonstrated here:
- [](https://huggingface.co/spaces/optimum/llm-perf-leaderboard)
- - *LLM Carbon Efficiency*: It appears that Hugging Face's datasheets do not currently include a specific metric for carbon efficiency. Instead, data on LLM energy efficiency, measured in tokens per kilowatt-hour (kWh), might be used as an indirect indicator of an LLM's carbon efficiency.
-
-- **Rating Algorithm**
- - Develop an algorithm to calculate efficiency scores, incorporating factors like efficiency under different loads and overall operational carbon footprint.
- - Hugging Face uses CodeCarbon to get energy consumed in kWh, which is then divided by the number of tokens generated
- - Rating Scale: The performance rating scale for Hugging Face's large language models (LLMs) ranges from 0 to 100. Currently, there is no specific scale for measuring energy efficiency. Hugging Face measures the energy efficiency of LLMs in terms of tokens per kilowatt-hour (kWh), rather than measuring carbon efficiency in CO2 equivalent per Unit of Software Function (USF) or computational output, e.g. carbon per 1000 tokens generated. However, if the benchmarking infrastructure used is consistent, then energy efficiency could be correlated with carbon efficiency.
-
-
- - Recommendations:
- 1. To effectively showcase the carbon efficiencies of LLMs, it is recommended to measure and present the CO2e emissions per x number of tokens generated.
- 2. Implement both a relative rating scale and absolute CO2e values: Calculate the ratings of each LLM's carbon efficiency among all the submitted LLMs in the same category. This approach will enable users to easily identify the most carbon-efficient LLM among those submitted in the same category.
-
-- **Compliance and Thresholds**
- - Set thresholds for ratings that align with industry standards or regulatory guidelines for energy efficiency.
- - At present, the industry lacks a clear consensus on establishing thresholds, let alone on compliance.
-
-### 4. Visualization and Labeling
-Visuals and labeling are crucial elements of the specification because a key objective is to facilitate the clear and intuitive presentation of an LLM's carbon efficiency. This enables users to easily understand and compare the carbon efficiency of different LLMs, helping them make well-informed decisions when selecting models.
-- **Design of Labels**
- - Create visually distinctive labels that clearly communicate the carbon efficiency rating of an LLM, similar to energy efficiency labels on appliances.
-- **Integration Points**
- - Specify how these labels will be integrated and displayed on platforms like Hugging Face or benchmarks like MLPerf, or if the rating is for an organization's internal consumption, choose an appropriate integration point.
-- **User Access and Transparency**
- - Ensure that the labels are easily accessible and understandable to users, providing detailed explanations of the ratings through tooltips or supplementary guides.
-
-## Observations
-### Gaps, Opportunities, and Observations
-
-- Currently, Hugging Face presents an extensive array of data for LLM benchmark results. The challenge lies in the usability of this raw data. Finding ways to simplify and clarify this vast amount of information to assist users in making informed decisions presents a significant opportunity for enhancement.
-- It seems that there is a trade-off between carbon efficiency and performance efficiency in large language models (LLMs). More accurate and high-performing LLMs, which often have billions of parameters, inherently require more energy for training and inference.
-- An effective strategy to reconcile performance with carbon efficiency could involve developing smaller, domain-specific LLMs. These models would be more carbon-efficient due to their reduced size but would remain highly performant and accurate by training exclusively on domain-specific data. This approach allows for maintaining the utility of large models where necessary, while also providing an option that requires less energy. Additionally, it suggests that such models should be evaluated and rated using distinct benchmarks tailored to their specific categories.
-### Potential Beneficiaries of Adopting SCER for LLMs Specification
-Any organization that evaluates or distributes LLMs (AI models) internally or externally can benefit from adopting the SCER for LLMs specification, because SCER specification and its certification enhance credibility, ensure regulatory compliance, attract sustainability-conscious consumers and stakeholders, and boost brand recognition.
-
-Governments and standard-setting bodies (SDOs) can greatly benefit from adopting the SCER for LLMs specification. For governments, these standards ensure industry-wide adherence to best practices in carbon efficiency for AI models used in public services and the broader industry, aligning with national and international sustainability goals. They also aid in regulatory oversight, enabling more effective monitoring and enforcement of sustainability practices in AI development.
-
-For SDOs, the SCER for LLMs specification offers a clear and consistent framework for evaluating the sustainability of LLMs, promoting uniformity and comparability across the industry. This facilitates the development of robust certification programs like the SCER Certification Program, enhances industry-wide carbon-efficient best practices, and drives innovation in sustainable AI technologies.
-
-## References
-
-### Sample Illustrations of SCER Rating - Visualization and Labeling
-
-
-
-
-
-
-### Nutri-Score:
-Nutri-Score is a front-of-package nutritional label that converts the nutritional value of food and beverages into a simple overall score. It is based on a scale of five colors and letters:
-- A: Green to represent the best nutritional quality
-- B: Light green, meaning it's still a favorable choice
-- C: Yellow, a balanced choice
-- D: Orange, less favorable
-- E: Dark orange to show it is the lowest
-
-The Nutri-Score calculation pinpoints the nutritional value of a product based on the ingredients. It takes into account both positive points (fiber content, protein, vegetables, fruit, and nuts) and negative points (kilojoules, fat, saturated fatty acids, sugar, and salt).
-
-The Nutri-Score is calculated per 100g or 100ml. The goal of the Nutri-Score is to influence consumers at the point of purchase to choose food products with a better nutritional profile, and to incentivize food manufacturers to improve the nutritional quality of their products.
-
-
-
-### Energy Guide and EnergyStar
-
-
-[**Energy Guide:**](https://consumer.ftc.gov/articles/how-use-energyguide-label-shop-home-appliances)
-The Energy Guide label is a prominent yellow label found on many home appliances. It provides consumers with essential information about the appliance’s energy consumption and efficiency, allowing them to compare the energy use of different models. The label includes estimates of annual energy use, operating costs, and how the appliance compares to similar models in terms of energy efficiency.
-
-
-
-
-[**Energy Star:**](https://www.energystar.gov/)
-Energy Star is a government-backed program and symbol for energy efficiency, managed by the U.S. Environmental Protection Agency (EPA). Products that earn the Energy Star label meet strict energy efficiency criteria set by the EPA, helping consumers save money on energy bills while reducing their environmental impact. Energy Star covers a wide range of products, including appliances, electronics, heating and cooling systems, and even entire buildings.
-
-
-
-
-
-### CDP:
-[CDP](https://www.cdp.net/en/info/about-us) (formerly Carbon Disclosure Project): A global disclosure system for companies, cities, states, and regions to manage their environmental impacts.
-- Data collection as a form of company disclosure: CDP provides guide that covers the key steps to disclose as a company including setting up a CDP account, responding to the CDP questionnaire(s), and receiving a CDP score.
-- A CDP score is a snapshot of a company’s environmental disclosure and performance. CDP's scoring methodology is fully aligned with regulatory boards and standards, and provides comparability in the market.
-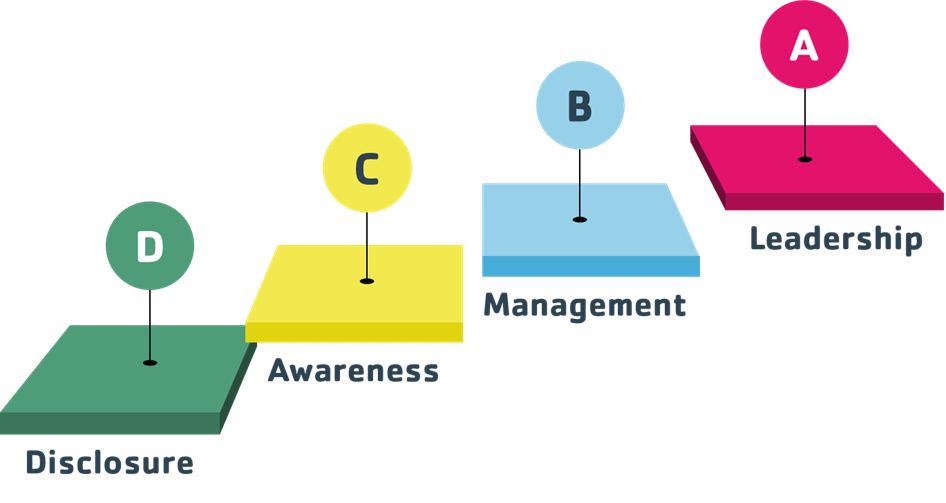
-
-
-### LEED
-LEED stands for Leadership in Energy and Environmental Design. It is the most widely used green building rating system in the world. LEED is an environmentally oriented building certification program run by the U.S. Green Building Council (USGBC).
-LEED provides a framework for healthy, efficient, and cost-saving green buildings. It aims to improve building and construction project performance across seven areas of environmental and human health.
-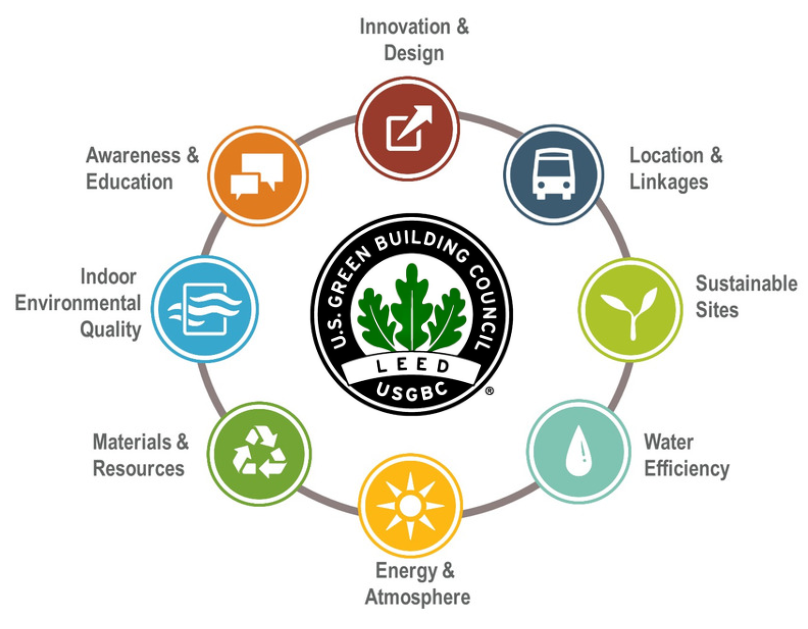
-
-To achieve LEED certification, a project earns points by adhering to prerequisites and credits that address carbon, energy, water, waste, transportation, materials, health and indoor environmental quality. Projects go through a verification and review process by GBCI and are awarded points that correspond to a level of LEED certification: **Certified (40-49 points), Silver (50-59 points), Gold (60-79 points) and Platinum (80+ points)**.
-
-
diff --git a/use_cases/SCER_FOR_LLM/images/average_score.png b/use_cases/SCER_FOR_LLM/images/average_score.png
deleted file mode 100644
index a4353e9..0000000
Binary files a/use_cases/SCER_FOR_LLM/images/average_score.png and /dev/null differ
diff --git a/use_cases/SCER_FOR_LLM/images/cc.jpg b/use_cases/SCER_FOR_LLM/images/cc.jpg
deleted file mode 100644
index 1422e6b..0000000
Binary files a/use_cases/SCER_FOR_LLM/images/cc.jpg and /dev/null differ
diff --git a/use_cases/SCER_FOR_LLM/images/image-1.png b/use_cases/SCER_FOR_LLM/images/image-1.png
deleted file mode 100644
index 109c4a2..0000000
Binary files a/use_cases/SCER_FOR_LLM/images/image-1.png and /dev/null differ
diff --git a/use_cases/SCER_FOR_LLM/images/image-2.png b/use_cases/SCER_FOR_LLM/images/image-2.png
deleted file mode 100644
index 191ea87..0000000
Binary files a/use_cases/SCER_FOR_LLM/images/image-2.png and /dev/null differ
diff --git a/use_cases/SCER_FOR_LLM/images/image-3.png b/use_cases/SCER_FOR_LLM/images/image-3.png
deleted file mode 100644
index 0ee56b4..0000000
Binary files a/use_cases/SCER_FOR_LLM/images/image-3.png and /dev/null differ
diff --git a/use_cases/SCER_FOR_LLM/images/image-4.png b/use_cases/SCER_FOR_LLM/images/image-4.png
deleted file mode 100644
index d91f134..0000000
Binary files a/use_cases/SCER_FOR_LLM/images/image-4.png and /dev/null differ
diff --git a/use_cases/SCER_FOR_LLM/images/image-5.png b/use_cases/SCER_FOR_LLM/images/image-5.png
deleted file mode 100644
index f445795..0000000
Binary files a/use_cases/SCER_FOR_LLM/images/image-5.png and /dev/null differ
diff --git a/use_cases/SCER_FOR_LLM/images/image-6.png b/use_cases/SCER_FOR_LLM/images/image-6.png
deleted file mode 100644
index 7061ba0..0000000
Binary files a/use_cases/SCER_FOR_LLM/images/image-6.png and /dev/null differ
diff --git a/use_cases/SCER_FOR_LLM/images/image-7.png b/use_cases/SCER_FOR_LLM/images/image-7.png
deleted file mode 100644
index c795fc6..0000000
Binary files a/use_cases/SCER_FOR_LLM/images/image-7.png and /dev/null differ
diff --git a/use_cases/SCER_FOR_LLM/images/image.png b/use_cases/SCER_FOR_LLM/images/image.png
deleted file mode 100644
index 9db2c09..0000000
Binary files a/use_cases/SCER_FOR_LLM/images/image.png and /dev/null differ
diff --git a/use_cases/SCER_FOR_LLM/images/tokensless.png b/use_cases/SCER_FOR_LLM/images/tokensless.png
deleted file mode 100644
index b4ec49c..0000000
Binary files a/use_cases/SCER_FOR_LLM/images/tokensless.png and /dev/null differ
diff --git a/use_cases/SCER_FOR_LLM/images/tokensmore.png b/use_cases/SCER_FOR_LLM/images/tokensmore.png
deleted file mode 100644
index d91c141..0000000
Binary files a/use_cases/SCER_FOR_LLM/images/tokensmore.png and /dev/null differ