diff --git a/site/en/tutorials/images/transfer_learning.ipynb b/site/en/tutorials/images/transfer_learning.ipynb
index 6406ccdce74..30353697208 100644
--- a/site/en/tutorials/images/transfer_learning.ipynb
+++ b/site/en/tutorials/images/transfer_learning.ipynb
@@ -585,7 +585,7 @@
},
"outputs": [],
"source": [
- "prediction_layer = tf.keras.layers.Dense(1)\n",
+ "prediction_layer = tf.keras.layers.Dense(1, activation='sigmoid')\n",
"prediction_batch = prediction_layer(feature_batch_average)\n",
"print(prediction_batch.shape)"
]
@@ -667,7 +667,7 @@
"source": [
"### Compile the model\n",
"\n",
- "Compile the model before training it. Since there are two classes, use the `tf.keras.losses.BinaryCrossentropy` loss with `from_logits=True` since the model provides a linear output."
+ "Compile the model before training it. Since there are two classes and a sigmoid oputput, use the `BinaryAccuracy`."
]
},
{
@@ -680,8 +680,8 @@
"source": [
"base_learning_rate = 0.0001\n",
"model.compile(optimizer=tf.keras.optimizers.Adam(learning_rate=base_learning_rate),\n",
- " loss=tf.keras.losses.BinaryCrossentropy(from_logits=True),\n",
- " metrics=[tf.keras.metrics.BinaryAccuracy(threshold=0, name='accuracy')])"
+ " loss=tf.keras.losses.BinaryCrossentropy(),\n",
+ " metrics=[tf.keras.metrics.BinaryAccuracy(threshold=0.5, name='accuracy')])"
]
},
{
@@ -872,9 +872,9 @@
},
"outputs": [],
"source": [
- "model.compile(loss=tf.keras.losses.BinaryCrossentropy(from_logits=True),\n",
+ "model.compile(loss=tf.keras.losses.BinaryCrossentropy(),\n",
" optimizer = tf.keras.optimizers.RMSprop(learning_rate=base_learning_rate/10),\n",
- " metrics=[tf.keras.metrics.BinaryAccuracy(threshold=0, name='accuracy')])"
+ " metrics=[tf.keras.metrics.BinaryAccuracy(threshold=0.5, name='accuracy')])"
]
},
{
@@ -1081,22 +1081,13 @@
"\n",
"To learn more, visit the [Transfer learning guide](https://www.tensorflow.org/guide/keras/transfer_learning).\n"
]
- },
- {
- "cell_type": "code",
- "execution_count": null,
- "metadata": {
- "id": "uKIByL01da8c"
- },
- "outputs": [],
- "source": []
}
],
"metadata": {
"accelerator": "GPU",
"colab": {
"name": "transfer_learning.ipynb",
- "private_outputs": true,
+ "provenance": [],
"toc_visible": true
},
"kernelspec": {
diff --git a/site/en/tutorials/keras/text_classification.ipynb b/site/en/tutorials/keras/text_classification.ipynb
index f14964207ff..c66d0fce0d3 100644
--- a/site/en/tutorials/keras/text_classification.ipynb
+++ b/site/en/tutorials/keras/text_classification.ipynb
@@ -267,9 +267,9 @@
"id": "95kkUdRoaeMw"
},
"source": [
- "Next, you will use the `text_dataset_from_directory` utility to create a labeled `tf.data.Dataset`. [tf.data](https://www.tensorflow.org/guide/data) is a powerful collection of tools for working with data. \n",
+ "Next, you will use the `text_dataset_from_directory` utility to create a labeled `tf.data.Dataset`. [tf.data](https://www.tensorflow.org/guide/data) is a powerful collection of tools for working with data.\n",
"\n",
- "When running a machine learning experiment, it is a best practice to divide your dataset into three splits: [train](https://developers.google.com/machine-learning/glossary#training_set), [validation](https://developers.google.com/machine-learning/glossary#validation_set), and [test](https://developers.google.com/machine-learning/glossary#test-set). \n",
+ "When running a machine learning experiment, it is a best practice to divide your dataset into three splits: [train](https://developers.google.com/machine-learning/glossary#training_set), [validation](https://developers.google.com/machine-learning/glossary#validation_set), and [test](https://developers.google.com/machine-learning/glossary#test-set).\n",
"\n",
"The IMDB dataset has already been divided into train and test, but it lacks a validation set. Let's create a validation set using an 80:20 split of the training data by using the `validation_split` argument below."
]
@@ -286,10 +286,10 @@
"seed = 42\n",
"\n",
"raw_train_ds = tf.keras.utils.text_dataset_from_directory(\n",
- " 'aclImdb/train', \n",
- " batch_size=batch_size, \n",
- " validation_split=0.2, \n",
- " subset='training', \n",
+ " 'aclImdb/train',\n",
+ " batch_size=batch_size,\n",
+ " validation_split=0.2,\n",
+ " subset='training',\n",
" seed=seed)"
]
},
@@ -322,7 +322,7 @@
"id": "JWq1SUIrp1a-"
},
"source": [
- "Notice the reviews contain raw text (with punctuation and occasional HTML tags like `
`). You will show how to handle these in the following section. \n",
+ "Notice the reviews contain raw text (with punctuation and occasional HTML tags like `
`). You will show how to handle these in the following section.\n",
"\n",
"The labels are 0 or 1. To see which of these correspond to positive and negative movie reviews, you can check the `class_names` property on the dataset.\n"
]
@@ -366,10 +366,10 @@
"outputs": [],
"source": [
"raw_val_ds = tf.keras.utils.text_dataset_from_directory(\n",
- " 'aclImdb/train', \n",
- " batch_size=batch_size, \n",
- " validation_split=0.2, \n",
- " subset='validation', \n",
+ " 'aclImdb/train',\n",
+ " batch_size=batch_size,\n",
+ " validation_split=0.2,\n",
+ " subset='validation',\n",
" seed=seed)"
]
},
@@ -382,7 +382,7 @@
"outputs": [],
"source": [
"raw_test_ds = tf.keras.utils.text_dataset_from_directory(\n",
- " 'aclImdb/test', \n",
+ " 'aclImdb/test',\n",
" batch_size=batch_size)"
]
},
@@ -394,7 +394,7 @@
"source": [
"### Prepare the dataset for training\n",
"\n",
- "Next, you will standardize, tokenize, and vectorize the data using the helpful `tf.keras.layers.TextVectorization` layer. \n",
+ "Next, you will standardize, tokenize, and vectorize the data using the helpful `tf.keras.layers.TextVectorization` layer.\n",
"\n",
"Standardization refers to preprocessing the text, typically to remove punctuation or HTML elements to simplify the dataset. Tokenization refers to splitting strings into tokens (for example, splitting a sentence into individual words, by splitting on whitespace). Vectorization refers to converting tokens into numbers so they can be fed into a neural network. All of these tasks can be accomplished with this layer.\n",
"\n",
@@ -580,7 +580,7 @@
"\n",
"`.cache()` keeps data in memory after it's loaded off disk. This will ensure the dataset does not become a bottleneck while training your model. If your dataset is too large to fit into memory, you can also use this method to create a performant on-disk cache, which is more efficient to read than many small files.\n",
"\n",
- "`.prefetch()` overlaps data preprocessing and model execution while training. \n",
+ "`.prefetch()` overlaps data preprocessing and model execution while training.\n",
"\n",
"You can learn more about both methods, as well as how to cache data to disk in the [data performance guide](https://www.tensorflow.org/guide/data_performance)."
]
@@ -635,7 +635,7 @@
" layers.Dropout(0.2),\n",
" layers.GlobalAveragePooling1D(),\n",
" layers.Dropout(0.2),\n",
- " layers.Dense(1)])\n",
+ " layers.Dense(1, activation='sigmoid')])\n",
"\n",
"model.summary()"
]
@@ -674,9 +674,9 @@
},
"outputs": [],
"source": [
- "model.compile(loss=losses.BinaryCrossentropy(from_logits=True),\n",
+ "model.compile(loss=losses.BinaryCrossentropy(),\n",
" optimizer='adam',\n",
- " metrics=tf.metrics.BinaryAccuracy(threshold=0.0))"
+ " metrics=[tf.metrics.BinaryAccuracy(threshold=0.5)])"
]
},
{
@@ -884,11 +884,11 @@
},
"outputs": [],
"source": [
- "examples = [\n",
+ "examples = tf.constant([\n",
" \"The movie was great!\",\n",
" \"The movie was okay.\",\n",
" \"The movie was terrible...\"\n",
- "]\n",
+ "])\n",
"\n",
"export_model.predict(examples)"
]
@@ -916,7 +916,7 @@
"\n",
"This tutorial showed how to train a binary classifier from scratch on the IMDB dataset. As an exercise, you can modify this notebook to train a multi-class classifier to predict the tag of a programming question on [Stack Overflow](http://stackoverflow.com/).\n",
"\n",
- "A [dataset](https://storage.googleapis.com/download.tensorflow.org/data/stack_overflow_16k.tar.gz) has been prepared for you to use containing the body of several thousand programming questions (for example, \"How can I sort a dictionary by value in Python?\") posted to Stack Overflow. Each of these is labeled with exactly one tag (either Python, CSharp, JavaScript, or Java). Your task is to take a question as input, and predict the appropriate tag, in this case, Python. \n",
+ "A [dataset](https://storage.googleapis.com/download.tensorflow.org/data/stack_overflow_16k.tar.gz) has been prepared for you to use containing the body of several thousand programming questions (for example, \"How can I sort a dictionary by value in Python?\") posted to Stack Overflow. Each of these is labeled with exactly one tag (either Python, CSharp, JavaScript, or Java). Your task is to take a question as input, and predict the appropriate tag, in this case, Python.\n",
"\n",
"The dataset you will work with contains several thousand questions extracted from the much larger public Stack Overflow dataset on [BigQuery](https://console.cloud.google.com/marketplace/details/stack-exchange/stack-overflow), which contains more than 17 million posts.\n",
"\n",
@@ -950,7 +950,7 @@
"\n",
"1. When plotting accuracy over time, change `binary_accuracy` and `val_binary_accuracy` to `accuracy` and `val_accuracy`, respectively.\n",
"\n",
- "1. Once these changes are complete, you will be able to train a multi-class classifier. "
+ "1. Once these changes are complete, you will be able to train a multi-class classifier."
]
},
{
@@ -968,8 +968,8 @@
"metadata": {
"accelerator": "GPU",
"colab": {
- "collapsed_sections": [],
"name": "text_classification.ipynb",
+ "provenance": [],
"toc_visible": true
},
"kernelspec": {
diff --git a/site/en/tutorials/quickstart/advanced.ipynb b/site/en/tutorials/quickstart/advanced.ipynb
index 2fe0ce85773..7cc134b2613 100644
--- a/site/en/tutorials/quickstart/advanced.ipynb
+++ b/site/en/tutorials/quickstart/advanced.ipynb
@@ -200,7 +200,7 @@
"id": "uGih-c2LgbJu"
},
"source": [
- "Choose an optimizer and loss function for training: "
+ "Choose an optimizer and loss function for training:"
]
},
{
@@ -311,10 +311,10 @@
"\n",
"for epoch in range(EPOCHS):\n",
" # Reset the metrics at the start of the next epoch\n",
- " train_loss.reset_states()\n",
- " train_accuracy.reset_states()\n",
- " test_loss.reset_states()\n",
- " test_accuracy.reset_states()\n",
+ " train_loss.reset_state()\n",
+ " train_accuracy.reset_state()\n",
+ " test_loss.reset_state()\n",
+ " test_accuracy.reset_state()\n",
"\n",
" for images, labels in train_ds:\n",
" train_step(images, labels)\n",
@@ -324,10 +324,10 @@
"\n",
" print(\n",
" f'Epoch {epoch + 1}, '\n",
- " f'Loss: {train_loss.result()}, '\n",
- " f'Accuracy: {train_accuracy.result() * 100}, '\n",
- " f'Test Loss: {test_loss.result()}, '\n",
- " f'Test Accuracy: {test_accuracy.result() * 100}'\n",
+ " f'Loss: {train_loss.result():0.2f}, '\n",
+ " f'Accuracy: {train_accuracy.result() * 100:0.2f}, '\n",
+ " f'Test Loss: {test_loss.result():0.2f}, '\n",
+ " f'Test Accuracy: {test_accuracy.result() * 100:0.2f}'\n",
" )"
]
},
@@ -344,8 +344,8 @@
"metadata": {
"accelerator": "GPU",
"colab": {
- "collapsed_sections": [],
"name": "advanced.ipynb",
+ "provenance": [],
"toc_visible": true
},
"kernelspec": {
diff --git a/site/en/tutorials/structured_data/time_series.ipynb b/site/en/tutorials/structured_data/time_series.ipynb
index 0b0eb55bce3..31aab384859 100644
--- a/site/en/tutorials/structured_data/time_series.ipynb
+++ b/site/en/tutorials/structured_data/time_series.ipynb
@@ -70,7 +70,7 @@
"source": [
"This tutorial is an introduction to time series forecasting using TensorFlow. It builds a few different styles of models including Convolutional and Recurrent Neural Networks (CNNs and RNNs).\n",
"\n",
- "This is covered in two main parts, with subsections: \n",
+ "This is covered in two main parts, with subsections:\n",
"\n",
"* Forecast for a single time step:\n",
" * A single feature.\n",
@@ -452,7 +452,7 @@
"id": "HiurzTGQgf_D"
},
"source": [
- "This gives the model access to the most important frequency features. In this case you knew ahead of time which frequencies were important. \n",
+ "This gives the model access to the most important frequency features. In this case you knew ahead of time which frequencies were important.\n",
"\n",
"If you don't have that information, you can determine which frequencies are important by extracting features with Fast Fourier Transform. To check the assumptions, here is the `tf.signal.rfft` of the temperature over time. Note the obvious peaks at frequencies near `1/year` and `1/day`:\n"
]
@@ -590,13 +590,13 @@
"source": [
"## Data windowing\n",
"\n",
- "The models in this tutorial will make a set of predictions based on a window of consecutive samples from the data. \n",
+ "The models in this tutorial will make a set of predictions based on a window of consecutive samples from the data.\n",
"\n",
"The main features of the input windows are:\n",
"\n",
"- The width (number of time steps) of the input and label windows.\n",
"- The time offset between them.\n",
- "- Which features are used as inputs, labels, or both. \n",
+ "- Which features are used as inputs, labels, or both.\n",
"\n",
"This tutorial builds a variety of models (including Linear, DNN, CNN and RNN models), and uses them for both:\n",
"\n",
@@ -616,11 +616,11 @@
"\n",
"1. For example, to make a single prediction 24 hours into the future, given 24 hours of history, you might define a window like this:\n",
"\n",
- " \n",
+ " 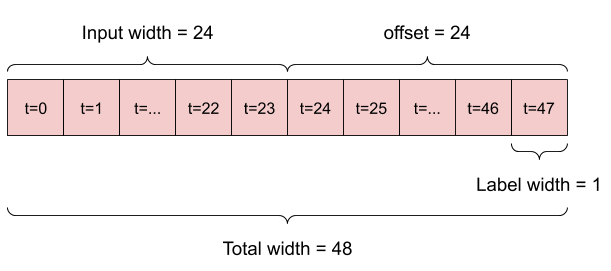\n",
"\n",
"2. A model that makes a prediction one hour into the future, given six hours of history, would need a window like this:\n",
"\n",
- " "
+ " 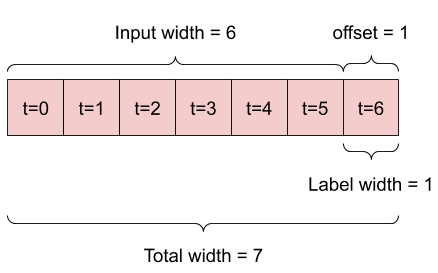"
]
},
{
@@ -744,7 +744,7 @@
"\n",
"The example `w2` you define earlier will be split like this:\n",
"\n",
- "\n",
+ "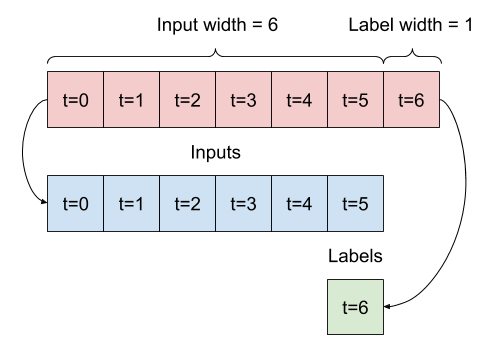\n",
"\n",
"This diagram doesn't show the `features` axis of the data, but this `split_window` function also handles the `label_columns` so it can be used for both the single output and multi-output examples."
]
@@ -1069,7 +1069,7 @@
"\n",
"So, start by building models to predict the `T (degC)` value one hour into the future.\n",
"\n",
- "\n",
+ "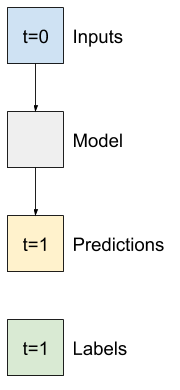\n",
"\n",
"Configure a `WindowGenerator` object to produce these single-step `(input, label)` pairs:"
]
@@ -1120,11 +1120,11 @@
"\n",
"Before building a trainable model it would be good to have a performance baseline as a point for comparison with the later more complicated models.\n",
"\n",
- "This first task is to predict temperature one hour into the future, given the current value of all features. The current values include the current temperature. \n",
+ "This first task is to predict temperature one hour into the future, given the current value of all features. The current values include the current temperature.\n",
"\n",
"So, start with a model that just returns the current temperature as the prediction, predicting \"No change\". This is a reasonable baseline since temperature changes slowly. Of course, this baseline will work less well if you make a prediction further in the future.\n",
"\n",
- ""
+ "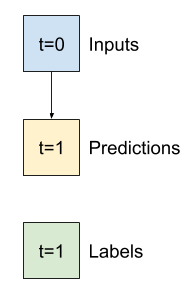"
]
},
{
@@ -1171,8 +1171,8 @@
"\n",
"val_performance = {}\n",
"performance = {}\n",
- "val_performance['Baseline'] = baseline.evaluate(single_step_window.val)\n",
- "performance['Baseline'] = baseline.evaluate(single_step_window.test, verbose=0)"
+ "val_performance['Baseline'] = baseline.evaluate(single_step_window.val, return_dict=True)\n",
+ "performance['Baseline'] = baseline.evaluate(single_step_window.test, verbose=0, return_dict=True)"
]
},
{
@@ -1211,7 +1211,7 @@
"source": [
"This expanded window can be passed directly to the same `baseline` model without any code changes. This is possible because the inputs and labels have the same number of time steps, and the baseline just forwards the input to the output:\n",
"\n",
- ""
+ "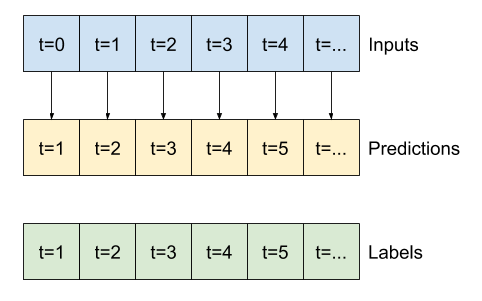"
]
},
{
@@ -1269,7 +1269,7 @@
"\n",
"The simplest **trainable** model you can apply to this task is to insert linear transformation between the input and output. In this case the output from a time step only depends on that step:\n",
"\n",
- "\n",
+ "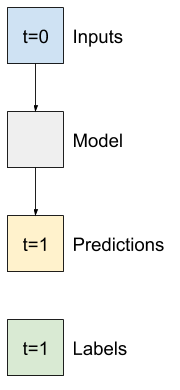\n",
"\n",
"A `tf.keras.layers.Dense` layer with no `activation` set is a linear model. The layer only transforms the last axis of the data from `(batch, time, inputs)` to `(batch, time, units)`; it is applied independently to every item across the `batch` and `time` axes."
]
@@ -1352,8 +1352,8 @@
"source": [
"history = compile_and_fit(linear, single_step_window)\n",
"\n",
- "val_performance['Linear'] = linear.evaluate(single_step_window.val)\n",
- "performance['Linear'] = linear.evaluate(single_step_window.test, verbose=0)"
+ "val_performance['Linear'] = linear.evaluate(single_step_window.val, return_dict=True)\n",
+ "performance['Linear'] = linear.evaluate(single_step_window.test, verbose=0, return_dict=True)"
]
},
{
@@ -1364,7 +1364,7 @@
"source": [
"Like the `baseline` model, the linear model can be called on batches of wide windows. Used this way the model makes a set of independent predictions on consecutive time steps. The `time` axis acts like another `batch` axis. There are no interactions between the predictions at each time step.\n",
"\n",
- ""
+ "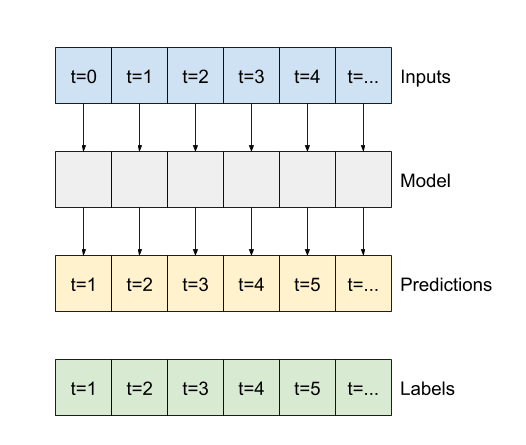"
]
},
{
@@ -1430,7 +1430,7 @@
"id": "Ylng7215boIY"
},
"source": [
- "Sometimes the model doesn't even place the most weight on the input `T (degC)`. This is one of the risks of random initialization. "
+ "Sometimes the model doesn't even place the most weight on the input `T (degC)`. This is one of the risks of random initialization."
]
},
{
@@ -1443,7 +1443,7 @@
"\n",
"Before applying models that actually operate on multiple time-steps, it's worth checking the performance of deeper, more powerful, single input step models.\n",
"\n",
- "Here's a model similar to the `linear` model, except it stacks several a few `Dense` layers between the input and the output: "
+ "Here's a model similar to the `linear` model, except it stacks several a few `Dense` layers between the input and the output:"
]
},
{
@@ -1462,8 +1462,8 @@
"\n",
"history = compile_and_fit(dense, single_step_window)\n",
"\n",
- "val_performance['Dense'] = dense.evaluate(single_step_window.val)\n",
- "performance['Dense'] = dense.evaluate(single_step_window.test, verbose=0)"
+ "val_performance['Dense'] = dense.evaluate(single_step_window.val, return_dict=True)\n",
+ "performance['Dense'] = dense.evaluate(single_step_window.test, verbose=0, return_dict=True)"
]
},
{
@@ -1476,7 +1476,7 @@
"\n",
"A single-time-step model has no context for the current values of its inputs. It can't see how the input features are changing over time. To address this issue the model needs access to multiple time steps when making predictions:\n",
"\n",
- "\n"
+ "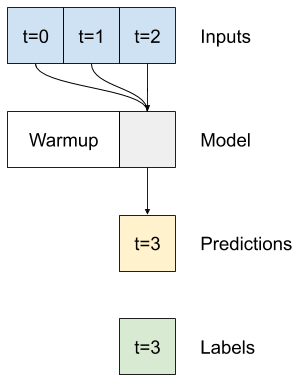\n"
]
},
{
@@ -1526,7 +1526,7 @@
"outputs": [],
"source": [
"conv_window.plot()\n",
- "plt.title(\"Given 3 hours of inputs, predict 1 hour into the future.\")"
+ "plt.suptitle(\"Given 3 hours of inputs, predict 1 hour into the future.\")"
]
},
{
@@ -1581,8 +1581,8 @@
"history = compile_and_fit(multi_step_dense, conv_window)\n",
"\n",
"IPython.display.clear_output()\n",
- "val_performance['Multi step dense'] = multi_step_dense.evaluate(conv_window.val)\n",
- "performance['Multi step dense'] = multi_step_dense.evaluate(conv_window.test, verbose=0)"
+ "val_performance['Multi step dense'] = multi_step_dense.evaluate(conv_window.val, return_dict=True)\n",
+ "performance['Multi step dense'] = multi_step_dense.evaluate(conv_window.test, verbose=0, return_dict=True)"
]
},
{
@@ -1602,7 +1602,7 @@
"id": "gWfrsP8mq8lV"
},
"source": [
- "The main down-side of this approach is that the resulting model can only be executed on input windows of exactly this shape. "
+ "The main down-side of this approach is that the resulting model can only be executed on input windows of exactly this shape."
]
},
{
@@ -1636,7 +1636,7 @@
},
"source": [
"### Convolution neural network\n",
- " \n",
+ "\n",
"A convolution layer (`tf.keras.layers.Conv1D`) also takes multiple time steps as input to each prediction."
]
},
@@ -1646,7 +1646,7 @@
"id": "cdLBwoaHmsWb"
},
"source": [
- "Below is the **same** model as `multi_step_dense`, re-written with a convolution. \n",
+ "Below is the **same** model as `multi_step_dense`, re-written with a convolution.\n",
"\n",
"Note the changes:\n",
"* The `tf.keras.layers.Flatten` and the first `tf.keras.layers.Dense` are replaced by a `tf.keras.layers.Conv1D`.\n",
@@ -1712,8 +1712,8 @@
"history = compile_and_fit(conv_model, conv_window)\n",
"\n",
"IPython.display.clear_output()\n",
- "val_performance['Conv'] = conv_model.evaluate(conv_window.val)\n",
- "performance['Conv'] = conv_model.evaluate(conv_window.test, verbose=0)"
+ "val_performance['Conv'] = conv_model.evaluate(conv_window.val, return_dict=True)\n",
+ "performance['Conv'] = conv_model.evaluate(conv_window.test, verbose=0, return_dict=True)"
]
},
{
@@ -1724,7 +1724,7 @@
"source": [
"The difference between this `conv_model` and the `multi_step_dense` model is that the `conv_model` can be run on inputs of any length. The convolutional layer is applied to a sliding window of inputs:\n",
"\n",
- "\n",
+ "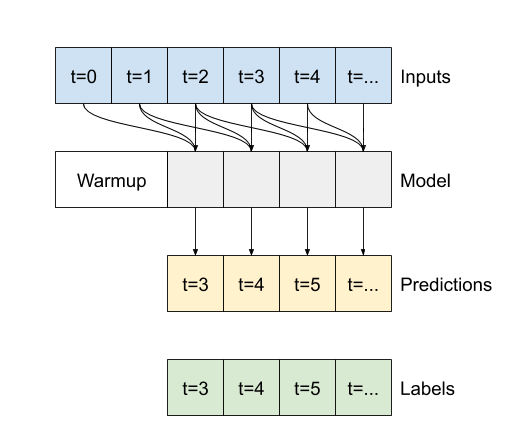\n",
"\n",
"If you run it on wider input, it produces wider output:"
]
@@ -1749,7 +1749,7 @@
"id": "h_WGxtLIHhRF"
},
"source": [
- "Note that the output is shorter than the input. To make training or plotting work, you need the labels, and prediction to have the same length. So build a `WindowGenerator` to produce wide windows with a few extra input time steps so the label and prediction lengths match: "
+ "Note that the output is shorter than the input. To make training or plotting work, you need the labels, and prediction to have the same length. So build a `WindowGenerator` to produce wide windows with a few extra input time steps so the label and prediction lengths match:"
]
},
{
@@ -1828,15 +1828,15 @@
"source": [
"An important constructor argument for all Keras RNN layers, such as `tf.keras.layers.LSTM`, is the `return_sequences` argument. This setting can configure the layer in one of two ways:\n",
"\n",
- "1. If `False`, the default, the layer only returns the output of the final time step, giving the model time to warm up its internal state before making a single prediction: \n",
+ "1. If `False`, the default, the layer only returns the output of the final time step, giving the model time to warm up its internal state before making a single prediction:\n",
"\n",
- "\n",
+ "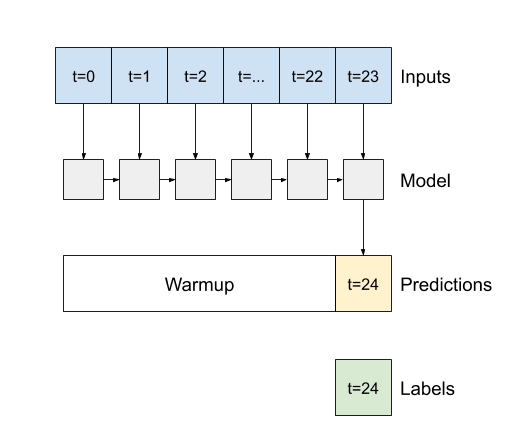\n",
"\n",
"2. If `True`, the layer returns an output for each input. This is useful for:\n",
- " * Stacking RNN layers. \n",
+ " * Stacking RNN layers.\n",
" * Training a model on multiple time steps simultaneously.\n",
"\n",
- ""
+ "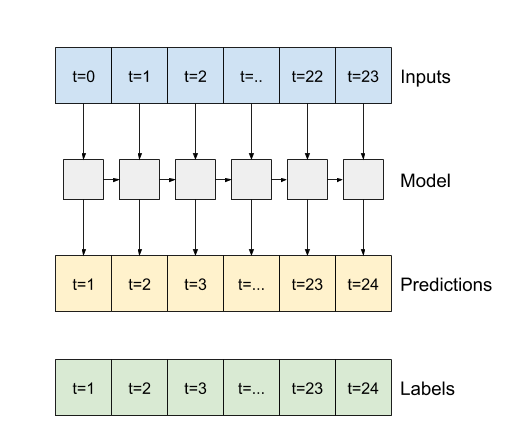"
]
},
{
@@ -1889,8 +1889,8 @@
"history = compile_and_fit(lstm_model, wide_window)\n",
"\n",
"IPython.display.clear_output()\n",
- "val_performance['LSTM'] = lstm_model.evaluate(wide_window.val)\n",
- "performance['LSTM'] = lstm_model.evaluate(wide_window.test, verbose=0)"
+ "val_performance['LSTM'] = lstm_model.evaluate(wide_window.val, return_dict=True)\n",
+ "performance['LSTM'] = lstm_model.evaluate(wide_window.test, verbose=0, return_dict=True)"
]
},
{
@@ -1922,6 +1922,29 @@
"With this dataset typically each of the models does slightly better than the one before it:"
]
},
+ {
+ "cell_type": "code",
+ "execution_count": null,
+ "metadata": {
+ "id": "dMPev9Nzd4mD"
+ },
+ "outputs": [],
+ "source": [
+ "cm = lstm_model.metrics[1]\n",
+ "cm.metrics"
+ ]
+ },
+ {
+ "cell_type": "code",
+ "execution_count": null,
+ "metadata": {
+ "id": "6is3g113eIIa"
+ },
+ "outputs": [],
+ "source": [
+ "val_performance"
+ ]
+ },
{
"cell_type": "code",
"execution_count": null,
@@ -1933,9 +1956,8 @@
"x = np.arange(len(performance))\n",
"width = 0.3\n",
"metric_name = 'mean_absolute_error'\n",
- "metric_index = lstm_model.metrics_names.index('mean_absolute_error')\n",
- "val_mae = [v[metric_index] for v in val_performance.values()]\n",
- "test_mae = [v[metric_index] for v in performance.values()]\n",
+ "val_mae = [v[metric_name] for v in val_performance.values()]\n",
+ "test_mae = [v[metric_name] for v in performance.values()]\n",
"\n",
"plt.ylabel('mean_absolute_error [T (degC), normalized]')\n",
"plt.bar(x - 0.17, val_mae, width, label='Validation')\n",
@@ -1954,7 +1976,7 @@
"outputs": [],
"source": [
"for name, value in performance.items():\n",
- " print(f'{name:12s}: {value[1]:0.4f}')"
+ " print(f'{name:12s}: {value[metric_name]:0.4f}')"
]
},
{
@@ -1979,7 +2001,7 @@
"outputs": [],
"source": [
"single_step_window = WindowGenerator(\n",
- " # `WindowGenerator` returns all features as labels if you \n",
+ " # `WindowGenerator` returns all features as labels if you\n",
" # don't set the `label_columns` argument.\n",
" input_width=1, label_width=1, shift=1)\n",
"\n",
@@ -2034,8 +2056,8 @@
"source": [
"val_performance = {}\n",
"performance = {}\n",
- "val_performance['Baseline'] = baseline.evaluate(wide_window.val)\n",
- "performance['Baseline'] = baseline.evaluate(wide_window.test, verbose=0)"
+ "val_performance['Baseline'] = baseline.evaluate(wide_window.val, return_dict=True)\n",
+ "performance['Baseline'] = baseline.evaluate(wide_window.test, verbose=0, return_dict=True)"
]
},
{
@@ -2073,8 +2095,8 @@
"history = compile_and_fit(dense, single_step_window)\n",
"\n",
"IPython.display.clear_output()\n",
- "val_performance['Dense'] = dense.evaluate(single_step_window.val)\n",
- "performance['Dense'] = dense.evaluate(single_step_window.test, verbose=0)"
+ "val_performance['Dense'] = dense.evaluate(single_step_window.val, return_dict=True)\n",
+ "performance['Dense'] = dense.evaluate(single_step_window.test, verbose=0, return_dict=True)"
]
},
{
@@ -2108,8 +2130,8 @@
"history = compile_and_fit(lstm_model, wide_window)\n",
"\n",
"IPython.display.clear_output()\n",
- "val_performance['LSTM'] = lstm_model.evaluate( wide_window.val)\n",
- "performance['LSTM'] = lstm_model.evaluate( wide_window.test, verbose=0)\n",
+ "val_performance['LSTM'] = lstm_model.evaluate( wide_window.val, return_dict=True)\n",
+ "performance['LSTM'] = lstm_model.evaluate( wide_window.test, verbose=0, return_dict=True)\n",
"\n",
"print()"
]
@@ -2132,7 +2154,7 @@
"\n",
"That is how you take advantage of the knowledge that the change should be small.\n",
"\n",
- "\n",
+ "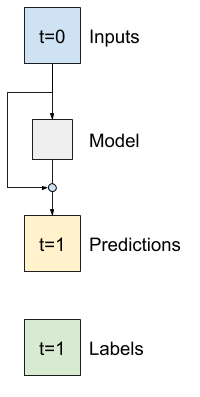\n",
"\n",
"Essentially, this initializes the model to match the `Baseline`. For this task it helps models converge faster, with slightly better performance."
]
@@ -2143,7 +2165,7 @@
"id": "yP58A_ORx0kM"
},
"source": [
- "This approach can be used in conjunction with any model discussed in this tutorial. \n",
+ "This approach can be used in conjunction with any model discussed in this tutorial.\n",
"\n",
"Here, it is being applied to the LSTM model, note the use of the `tf.initializers.zeros` to ensure that the initial predicted changes are small, and don't overpower the residual connection. There are no symmetry-breaking concerns for the gradients here, since the `zeros` are only used on the last layer."
]
@@ -2192,8 +2214,8 @@
"history = compile_and_fit(residual_lstm, wide_window)\n",
"\n",
"IPython.display.clear_output()\n",
- "val_performance['Residual LSTM'] = residual_lstm.evaluate(wide_window.val)\n",
- "performance['Residual LSTM'] = residual_lstm.evaluate(wide_window.test, verbose=0)\n",
+ "val_performance['Residual LSTM'] = residual_lstm.evaluate(wide_window.val, return_dict=True)\n",
+ "performance['Residual LSTM'] = residual_lstm.evaluate(wide_window.test, verbose=0, return_dict=True)\n",
"print()"
]
},
@@ -2227,9 +2249,8 @@
"width = 0.3\n",
"\n",
"metric_name = 'mean_absolute_error'\n",
- "metric_index = lstm_model.metrics_names.index('mean_absolute_error')\n",
- "val_mae = [v[metric_index] for v in val_performance.values()]\n",
- "test_mae = [v[metric_index] for v in performance.values()]\n",
+ "val_mae = [v[metric_name] for v in val_performance.values()]\n",
+ "test_mae = [v[metric_name] for v in performance.values()]\n",
"\n",
"plt.bar(x - 0.17, val_mae, width, label='Validation')\n",
"plt.bar(x + 0.17, test_mae, width, label='Test')\n",
@@ -2248,7 +2269,7 @@
"outputs": [],
"source": [
"for name, value in performance.items():\n",
- " print(f'{name:15s}: {value[1]:0.4f}')"
+ " print(f'{name:15s}: {value[metric_name]:0.4f}')"
]
},
{
@@ -2327,7 +2348,7 @@
"source": [
"A simple baseline for this task is to repeat the last input time step for the required number of output time steps:\n",
"\n",
- ""
+ "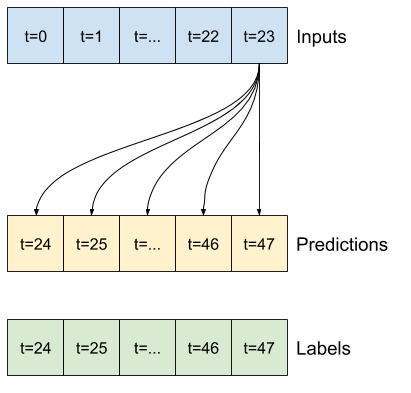"
]
},
{
@@ -2349,8 +2370,8 @@
"multi_val_performance = {}\n",
"multi_performance = {}\n",
"\n",
- "multi_val_performance['Last'] = last_baseline.evaluate(multi_window.val)\n",
- "multi_performance['Last'] = last_baseline.evaluate(multi_window.test, verbose=0)\n",
+ "multi_val_performance['Last'] = last_baseline.evaluate(multi_window.val, return_dict=True)\n",
+ "multi_performance['Last'] = last_baseline.evaluate(multi_window.test, verbose=0, return_dict=True)\n",
"multi_window.plot(last_baseline)"
]
},
@@ -2362,7 +2383,7 @@
"source": [
"Since this task is to predict 24 hours into the future, given 24 hours of the past, another simple approach is to repeat the previous day, assuming tomorrow will be similar:\n",
"\n",
- ""
+ "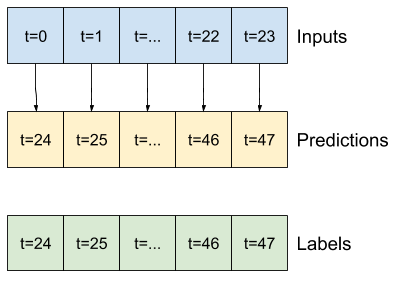"
]
},
{
@@ -2381,8 +2402,8 @@
"repeat_baseline.compile(loss=tf.keras.losses.MeanSquaredError(),\n",
" metrics=[tf.keras.metrics.MeanAbsoluteError()])\n",
"\n",
- "multi_val_performance['Repeat'] = repeat_baseline.evaluate(multi_window.val)\n",
- "multi_performance['Repeat'] = repeat_baseline.evaluate(multi_window.test, verbose=0)\n",
+ "multi_val_performance['Repeat'] = repeat_baseline.evaluate(multi_window.val, return_dict=True)\n",
+ "multi_performance['Repeat'] = repeat_baseline.evaluate(multi_window.test, verbose=0, return_dict=True)\n",
"multi_window.plot(repeat_baseline)"
]
},
@@ -2409,7 +2430,7 @@
"\n",
"A simple linear model based on the last input time step does better than either baseline, but is underpowered. The model needs to predict `OUTPUT_STEPS` time steps, from a single input time step with a linear projection. It can only capture a low-dimensional slice of the behavior, likely based mainly on the time of day and time of year.\n",
"\n",
- ""
+ "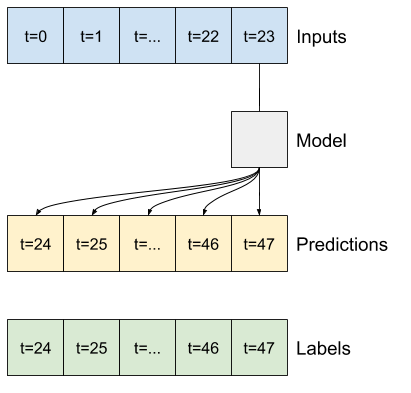"
]
},
{
@@ -2434,8 +2455,8 @@
"history = compile_and_fit(multi_linear_model, multi_window)\n",
"\n",
"IPython.display.clear_output()\n",
- "multi_val_performance['Linear'] = multi_linear_model.evaluate(multi_window.val)\n",
- "multi_performance['Linear'] = multi_linear_model.evaluate(multi_window.test, verbose=0)\n",
+ "multi_val_performance['Linear'] = multi_linear_model.evaluate(multi_window.val, return_dict=True)\n",
+ "multi_performance['Linear'] = multi_linear_model.evaluate(multi_window.test, verbose=0, return_dict=True)\n",
"multi_window.plot(multi_linear_model)"
]
},
@@ -2474,8 +2495,8 @@
"history = compile_and_fit(multi_dense_model, multi_window)\n",
"\n",
"IPython.display.clear_output()\n",
- "multi_val_performance['Dense'] = multi_dense_model.evaluate(multi_window.val)\n",
- "multi_performance['Dense'] = multi_dense_model.evaluate(multi_window.test, verbose=0)\n",
+ "multi_val_performance['Dense'] = multi_dense_model.evaluate(multi_window.val, return_dict=True)\n",
+ "multi_performance['Dense'] = multi_dense_model.evaluate(multi_window.test, verbose=0, return_dict=True)\n",
"multi_window.plot(multi_dense_model)"
]
},
@@ -2496,7 +2517,7 @@
"source": [
"A convolutional model makes predictions based on a fixed-width history, which may lead to better performance than the dense model since it can see how things are changing over time:\n",
"\n",
- ""
+ "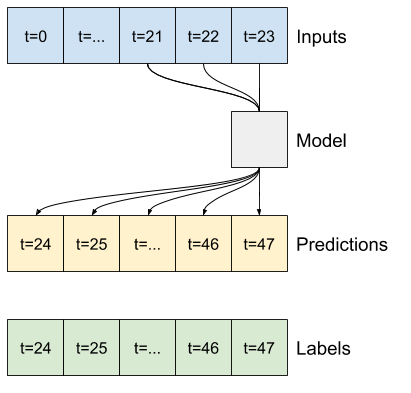"
]
},
{
@@ -2524,8 +2545,8 @@
"\n",
"IPython.display.clear_output()\n",
"\n",
- "multi_val_performance['Conv'] = multi_conv_model.evaluate(multi_window.val)\n",
- "multi_performance['Conv'] = multi_conv_model.evaluate(multi_window.test, verbose=0)\n",
+ "multi_val_performance['Conv'] = multi_conv_model.evaluate(multi_window.val, return_dict=True)\n",
+ "multi_performance['Conv'] = multi_conv_model.evaluate(multi_window.test, verbose=0, return_dict=True)\n",
"multi_window.plot(multi_conv_model)"
]
},
@@ -2548,7 +2569,7 @@
"\n",
"In this single-shot format, the LSTM only needs to produce an output at the last time step, so set `return_sequences=False` in `tf.keras.layers.LSTM`.\n",
"\n",
- "\n"
+ "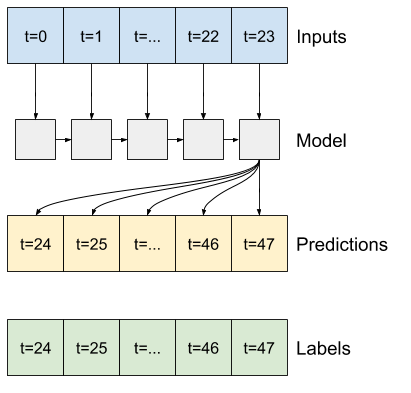\n"
]
},
{
@@ -2574,8 +2595,8 @@
"\n",
"IPython.display.clear_output()\n",
"\n",
- "multi_val_performance['LSTM'] = multi_lstm_model.evaluate(multi_window.val)\n",
- "multi_performance['LSTM'] = multi_lstm_model.evaluate(multi_window.test, verbose=0)\n",
+ "multi_val_performance['LSTM'] = multi_lstm_model.evaluate(multi_window.val, return_dict=True)\n",
+ "multi_performance['LSTM'] = multi_lstm_model.evaluate(multi_window.test, verbose=0, return_dict=True)\n",
"multi_window.plot(multi_lstm_model)"
]
},
@@ -2595,7 +2616,7 @@
"\n",
"You could take any of the single-step multi-output models trained in the first half of this tutorial and run in an autoregressive feedback loop, but here you'll focus on building a model that's been explicitly trained to do that.\n",
"\n",
- ""
+ "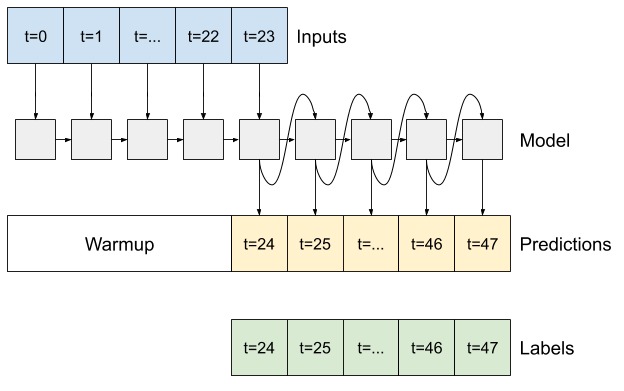"
]
},
{
@@ -2794,8 +2815,8 @@
"\n",
"IPython.display.clear_output()\n",
"\n",
- "multi_val_performance['AR LSTM'] = feedback_model.evaluate(multi_window.val)\n",
- "multi_performance['AR LSTM'] = feedback_model.evaluate(multi_window.test, verbose=0)\n",
+ "multi_val_performance['AR LSTM'] = feedback_model.evaluate(multi_window.val, return_dict=True)\n",
+ "multi_performance['AR LSTM'] = feedback_model.evaluate(multi_window.test, verbose=0, return_dict=True)\n",
"multi_window.plot(feedback_model)"
]
},
@@ -2829,9 +2850,8 @@
"width = 0.3\n",
"\n",
"metric_name = 'mean_absolute_error'\n",
- "metric_index = lstm_model.metrics_names.index('mean_absolute_error')\n",
- "val_mae = [v[metric_index] for v in multi_val_performance.values()]\n",
- "test_mae = [v[metric_index] for v in multi_performance.values()]\n",
+ "val_mae = [v[metric_name] for v in multi_val_performance.values()]\n",
+ "test_mae = [v[metric_name] for v in multi_performance.values()]\n",
"\n",
"plt.bar(x - 0.17, val_mae, width, label='Validation')\n",
"plt.bar(x + 0.17, test_mae, width, label='Test')\n",
@@ -2847,7 +2867,7 @@
"id": "Zq3hUsedCEmJ"
},
"source": [
- "The metrics for the multi-output models in the first half of this tutorial show the performance averaged across all output features. These performances are similar but also averaged across output time steps. "
+ "The metrics for the multi-output models in the first half of this tutorial show the performance averaged across all output features. These performances are similar but also averaged across output time steps."
]
},
{
@@ -2859,7 +2879,7 @@
"outputs": [],
"source": [
"for name, value in multi_performance.items():\n",
- " print(f'{name:8s}: {value[1]:0.4f}')"
+ " print(f'{name:8s}: {value[metric_name]:0.4f}')"
]
},
{
@@ -2894,8 +2914,8 @@
"metadata": {
"accelerator": "GPU",
"colab": {
- "collapsed_sections": [],
"name": "time_series.ipynb",
+ "provenance": [],
"toc_visible": true
},
"kernelspec": {